In recent years, there has been a significant advancement in the field of Artificial Intelligence (AI) and Augmented Reality (AR). These technologies have become increasingly popular and have the potential to enhance virtual experiences in various fields such as gaming, education, healthcare, and...
Advancements in AI Drug Discovery: Molecular Simulation, Synthesis Prediction, Trial Modeling
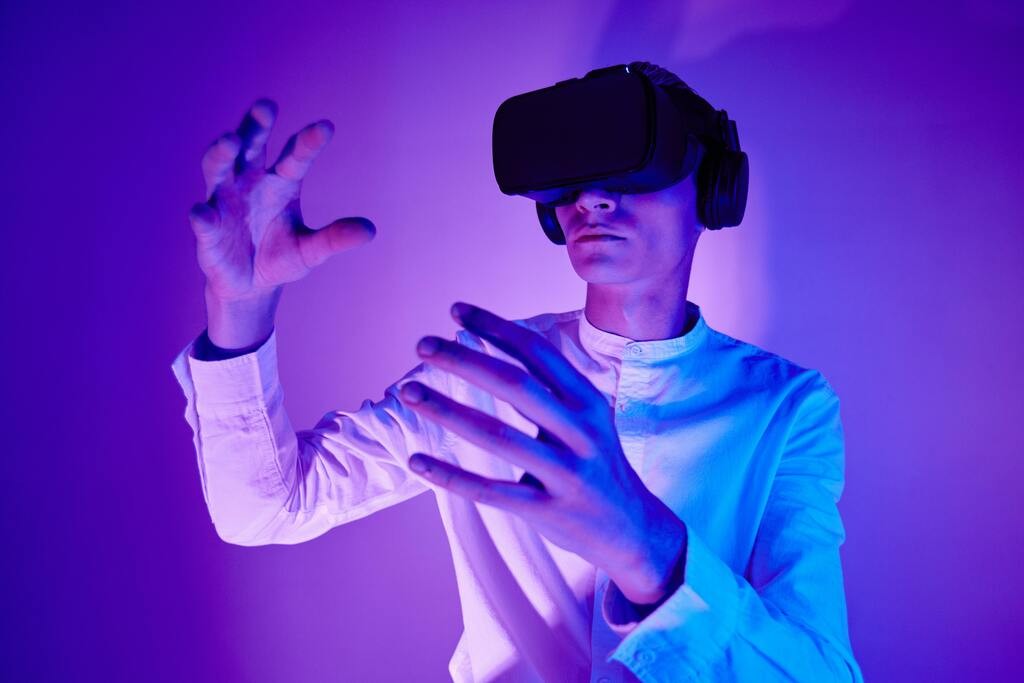
The field of drug discovery has seen significant advancements in recent years, thanks to the application of artificial intelligence (AI) technologies. These cutting-edge tools have revolutionized the way scientists approach the development of new drugs, making the process faster, more efficient, and more cost-effective.
One area where AI has made a tremendous impact is in molecular simulation. By using complex algorithms and machine learning models, researchers can now simulate the behavior of molecules and predict their interactions with target proteins. This allows them to identify potential drug candidates and optimize their properties before even stepping into the laboratory.
Another exciting development is the use of AI in synthesis prediction. Traditionally, chemists have relied on their intuition and experience to design synthetic routes for new compounds. However, with the help of AI, they can now leverage vast amounts of data to predict the most efficient and cost-effective ways to synthesize a desired molecule. This not only saves time and resources but also opens up new possibilities for drug discovery.
Furthermore, AI is also being utilized in trial modeling to improve the success rate of clinical trials. By analyzing vast amounts of patient data and clinical trial results, AI algorithms can identify patterns and make predictions about drug efficacy and safety. This allows researchers to design more targeted and personalized trials, increasing the chances of success and reducing the risk of adverse effects.
In conclusion, the advancements in AI drug discovery, particularly in molecular simulation, synthesis prediction, and trial modeling, have revolutionized the field. These technologies have the potential to accelerate the development of new drugs, reduce costs, and improve patient outcomes. As AI continues to evolve, we can expect even more exciting breakthroughs in the future.
Advancements in AI Drug Discovery
AI has revolutionized the field of drug discovery, enabling scientists to accelerate the process of identifying potential therapeutics and bringing them to market more efficiently. By leveraging machine learning algorithms and molecular simulations, AI has the potential to transform every stage of the drug development pipeline.
Molecular Simulation
Molecular simulation is a key focus area in AI drug discovery. It involves using computational models to simulate the behavior of molecules and predict their interactions with target proteins. By analyzing the molecular dynamics and energy landscapes, scientists can gain insights into the structure-activity relationships of potential drug candidates. This helps in identifying molecules with high binding affinity and selectivity, which are critical for designing effective therapeutics.
AI algorithms can analyze vast amounts of molecular data and identify patterns that are difficult for humans to detect. This has led to the development of more accurate and efficient simulation methods, such as molecular docking and molecular dynamics simulations. These techniques enable researchers to screen large libraries of compounds and prioritize the most promising candidates for further testing.
Synthesis Prediction
Another area where AI has made significant advancements in drug discovery is synthesis prediction. Predicting the synthesis routes of potential drug candidates is crucial for optimizing the production process and reducing costs. AI models can analyze chemical reaction databases and learn the rules governing organic synthesis. This enables them to predict the most efficient and cost-effective routes for synthesizing target molecules.
By leveraging AI, scientists can explore a wider range of synthesis possibilities and identify novel pathways that might have been overlooked using traditional methods. This not only speeds up the drug discovery process but also enables the synthesis of complex molecules that were previously considered impractical or too expensive.
AI-driven synthesis prediction has the potential to revolutionize the field of drug discovery by enabling the rapid and cost-effective synthesis of new therapeutics.
Trial Modeling
AI is also being used to improve the design and optimization of clinical trials. By analyzing large datasets from previous trials, AI models can identify patient populations that are more likely to respond positively to a particular treatment. This helps in designing more targeted and efficient clinical trials, reducing costs and time-to-market.
AI can also help in modeling the effects of different dosages and treatment regimens, allowing researchers to optimize the drug dosage and administration schedule. This can improve the efficacy and safety of the drug, while minimizing side effects.
Overall, AI is transforming the field of drug discovery by enabling faster and more efficient identification of potential therapeutics, optimizing synthesis routes, and improving the design of clinical trials.
Molecular Simulation
Molecular simulation is a powerful tool in the field of drug discovery, allowing scientists to study and understand the behavior of molecules at a microscopic level. By using computer algorithms and mathematical models, researchers can simulate the interactions between atoms and predict the properties and behavior of complex molecules.
Types of Molecular Simulation
There are several types of molecular simulations that are commonly used in drug discovery:
- Molecular Dynamics Simulation: This type of simulation tracks the positions and velocities of atoms over time, allowing researchers to study how molecules move and interact. It can provide insights into the stability, flexibility, and conformational changes of molecules.
- Monte Carlo Simulation: Monte Carlo simulations use statistical sampling techniques to explore the conformational space of molecules. It is particularly useful in predicting the thermodynamic properties of molecules, such as binding affinities and solubilities.
- Quantum Mechanics Simulation: Quantum mechanics simulations use quantum mechanical equations to calculate the electronic structure and properties of molecules. This type of simulation is especially valuable in understanding the reactivity and energy profiles of chemical reactions.
Applications of Molecular Simulation in Drug Discovery
Molecular simulation has a wide range of applications in drug discovery:
- Virtual Screening: By simulating the interactions between small molecules and target proteins, researchers can identify potential drug candidates. Virtual screening can significantly speed up the process of drug discovery by narrowing down the number of compounds that need to be tested experimentally.
- Lead Optimization: Molecular simulation can aid in the optimization of lead compounds by predicting their binding affinities and identifying potential modifications to enhance their potency and selectivity. This can help researchers design more effective and safer drugs.
- Drug Repurposing: By simulating the interactions between known drugs and different target proteins, researchers can identify new therapeutic uses for existing drugs. This approach can save time and resources by repurposing drugs that have already undergone extensive testing.
- ADME/Tox Prediction: Molecular simulation can predict the absorption, distribution, metabolism, excretion, and toxicity (ADME/Tox) properties of compounds. This information is crucial in the early stages of drug development to prioritize compounds with favorable pharmacokinetic and safety profiles.
In conclusion, molecular simulation is a valuable tool in the field of drug discovery, enabling researchers to gain insights into the behavior and properties of molecules. By leveraging the power of artificial intelligence and computational methods, scientists can accelerate the process of drug development and design more effective and safer drugs.
Synthesis Prediction
Synthesis prediction is a crucial aspect of AI drug discovery that involves using machine learning algorithms to predict the feasibility and optimal conditions for synthesizing new molecules. By analyzing existing data on known reactions and chemical structures, AI models can learn patterns and relationships that enable them to make accurate predictions about the synthesis of novel compounds.
One approach to synthesis prediction is to use graph neural networks (GNNs), which are capable of capturing the complex relationships between atoms and bonds in a molecule. GNNs can be trained on large datasets of known reactions to learn the rules and patterns that govern chemical transformations. By applying these learned rules to new molecules, GNNs can predict the most likely synthetic pathways and identify potential challenges or bottlenecks.
Another method for synthesis prediction is retrosynthesis, which involves working backwards from a target molecule to identify the necessary precursors and steps for its synthesis. AI models can be trained on databases of known reactions to learn the transformations and conditions required for specific chemical reactions. By applying these learned rules in reverse, AI models can suggest potential precursors and reaction pathways for synthesizing a desired molecule.
Synthesis prediction has the potential to greatly accelerate the drug discovery process by reducing the need for trial and error in the laboratory. By accurately predicting the synthesis of new compounds, researchers can prioritize the most promising candidates and focus their experimental efforts on the molecules with the highest likelihood of success. This not only saves time and resources but also increases the chances of discovering new drugs and therapies.
Trial Modeling
Trial modeling is a critical component of AI drug discovery, as it allows researchers to simulate and predict the outcomes of clinical trials for new drugs. By leveraging machine learning algorithms and large datasets, trial modeling can help identify potential issues and challenges that may arise during the drug development process.
One of the main advantages of trial modeling is its ability to save time and resources. Traditional clinical trials can be lengthy and expensive, often requiring years of testing and millions of dollars. By using AI algorithms, researchers can simulate a variety of scenarios and predict the potential efficacy and safety of a drug before conducting actual trials.
Additionally, trial modeling can help identify patient populations that are most likely to benefit from a new drug. By analyzing large datasets and incorporating factors such as demographics, genetics, and medical history, researchers can tailor their trials to specific groups, increasing the chances of success and reducing the risk of adverse events.
Furthermore, trial modeling can provide insights into the potential side effects and interactions of a new drug. By simulating the effects of a drug on various biological systems and predicting its interactions with other medications, researchers can better understand the potential risks and benefits before conducting trials.
- Improved efficacy prediction: Trial modeling can help predict the efficacy of a new drug by simulating its effects on targeted biological pathways and identifying potential therapeutic targets.
- Optimized trial design: By simulating various trial designs and scenarios, researchers can optimize the design of clinical trials, reducing costs and improving efficiency.
- Early identification of safety concerns: Trial modeling can help identify potential safety concerns and side effects of a drug before conducting trials, reducing the risk to patients.
- Enhanced decision-making: By providing researchers with valuable insights and predictions, trial modeling can help inform decision-making throughout the drug development process.
In conclusion, trial modeling is an essential tool in AI drug discovery. It enables researchers to simulate and predict the outcomes of clinical trials, saving time and resources, identifying patient populations that are most likely to benefit, and providing insights into potential side effects and interactions. With continued advancements in AI technology, trial modeling will play an increasingly important role in accelerating the development of new and effective drugs.