In recent years, there has been a significant advancement in the field of Artificial Intelligence (AI) and Augmented Reality (AR). These technologies have become increasingly popular and have the potential to enhance virtual experiences in various fields such as gaming, education, healthcare, and...
AI Drug Discovery - Unlocking the Potential of Molecular Simulation, Synthesis Prediction, and Trial Modeling
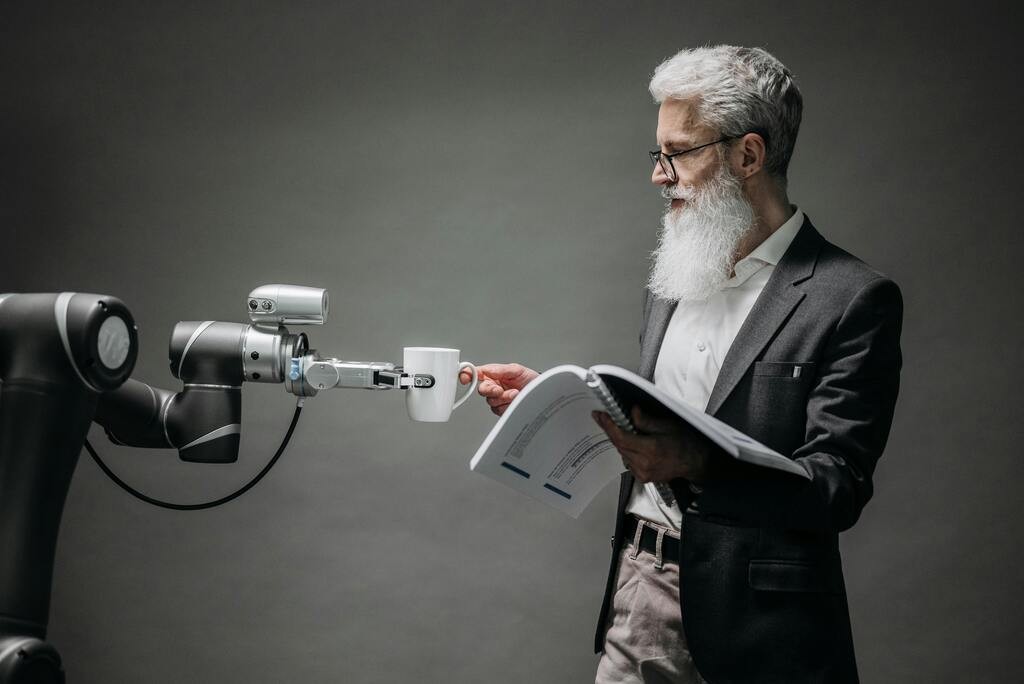
Advances in artificial intelligence (AI) have revolutionized various industries, and the field of drug discovery is no exception. With the ability to analyze vast amounts of data and simulate complex molecular interactions, AI has transformed the way scientists approach the development of new drugs. By leveraging machine learning algorithms and predictive modeling techniques, researchers can now expedite the process of identifying potential drug candidates, predicting their synthesis pathways, and modeling their efficacy in clinical trials.
Molecular simulation, a key aspect of drug discovery, involves studying the behavior and interactions of molecules in silico. Traditionally, this process required laborious and time-consuming experiments. However, with the advent of AI, scientists can now use algorithms to simulate and predict the behavior of molecules, saving both time and resources. Machine learning algorithms can analyze large datasets of molecular structures and properties to identify patterns and make predictions about the behavior of similar molecules. This enables researchers to screen thousands of potential drug candidates and prioritize the most promising ones for further investigation.
In addition to molecular simulation, AI is also revolutionizing the prediction of synthesis pathways. Developing new drugs often involves complex chemical reactions, and predicting the optimal synthesis pathway can be challenging. AI algorithms can analyze existing data on chemical reactions and propose novel synthesis routes based on this knowledge. By considering factors such as reaction efficiency and cost, AI can suggest the most efficient and cost-effective synthesis pathways, saving researchers valuable time and resources.
Furthermore, AI is transforming the modeling of clinical trials, a crucial step in drug development. Predicting the efficacy of a drug in a clinical trial is a complex task that requires considering various factors, such as the drug's mechanism of action and the characteristics of the patient population. AI algorithms can analyze diverse datasets, including genetic information, patient demographics, and historical trial data, to model the outcomes of clinical trials. This predictive modeling enables researchers to optimize trial design, identify potential risks, and make informed decisions about the progression of drug candidates through the clinical trial process.
In conclusion, AI is revolutionizing the field of drug discovery by enabling scientists to simulate molecular interactions, predict synthesis pathways, and model the outcomes of clinical trials. By leveraging machine learning algorithms and predictive modeling techniques, researchers can accelerate the development of new drugs and optimize the drug discovery process. With AI as a powerful tool, the future of drug discovery holds immense potential for the development of life-saving medications.
AI Drug Discovery: Revolutionizing Molecular Simulation
Molecular simulation is a crucial aspect of drug discovery, providing insights into the behavior of molecules and their interactions with target proteins. Traditionally, molecular simulation has been a time-consuming and resource-intensive process, requiring extensive computational power and expertise in physics and chemistry.
However, with the advent of artificial intelligence (AI), the landscape of molecular simulation has undergone a paradigm shift. AI has revolutionized the field by enabling more efficient and accurate simulations, thereby accelerating the drug discovery process.
One of the key applications of AI in molecular simulation is the prediction of molecular structures and properties. AI models can analyze vast amounts of data and learn patterns, allowing for the generation of accurate molecular structures and properties in a fraction of the time it would take using traditional methods.
Another area where AI has made significant contributions is in the prediction of molecular interactions. By training AI models on large datasets of known molecular interactions, researchers can now identify potential drug targets and design molecules that are more likely to interact with these targets. This enables the development of drugs with higher efficacy and fewer side effects.
In addition to prediction, AI has also enhanced the efficiency of molecular simulation through optimization algorithms. These algorithms can optimize the parameters of a simulation to maximize its accuracy and speed, allowing researchers to obtain results faster and with greater precision.
Furthermore, AI has facilitated the integration of multi-scale modeling approaches, which combine different levels of molecular detail to simulate complex biological systems. By incorporating AI into these models, researchers can better understand the behavior of drugs in the human body and predict their efficacy and safety.
Overall, AI has revolutionized molecular simulation in drug discovery, enabling faster and more accurate predictions of molecular structures, properties, and interactions. With further advancements in AI technology, the field of drug discovery is poised to make even greater strides in the future.
Enhancing Drug Design and Development Process
The advancement of artificial intelligence (AI) has brought about a significant transformation in the field of drug design and development. AI-powered tools and algorithms are revolutionizing the way drugs are discovered, designed, and tested, expediting the entire process and increasing the success rate of drug development.
AI is enhancing the drug design process by leveraging the power of molecular simulations. Traditional methods of drug discovery involved time-consuming and expensive laboratory experiments. However, AI-powered molecular simulations enable researchers to virtually predict the behavior and interactions of molecules, saving both time and resources. These simulations provide valuable insights into the molecular structure of potential drug candidates, helping researchers make informed decisions about their efficacy and safety.
Moreover, AI is playing a crucial role in predicting the synthesis of new molecules. By analyzing vast amounts of chemical data, AI algorithms can identify patterns and relationships that humans might miss. This enables scientists to predict the most efficient and cost-effective synthesis routes, accelerating the development of new drugs. With AI, researchers can explore a wider range of chemical space, leading to the discovery of novel drug candidates that were previously overlooked.
In addition to molecular simulation and synthesis prediction, AI is also revolutionizing the modeling of clinical trials. By analyzing large datasets from clinical trials, AI algorithms can identify patterns and correlations that can help optimize trial design and patient selection. This leads to more efficient and targeted trials, reducing costs and expediting the drug development process.
Overall, the integration of AI in drug design and development is transforming the pharmaceutical industry. AI-powered tools are enabling researchers to make more informed decisions, optimize processes, and discover new drug candidates more efficiently. With continued advancements in AI technology, the future of drug design and development looks promising, with the potential to revolutionize healthcare and improve patient outcomes.
Synthesis Prediction: AI-driven Advances
One of the most challenging aspects of drug discovery is predicting the synthesis of a potential drug molecule. Traditionally, chemists rely on their expertise and intuition to design and optimize synthetic routes. However, with the advent of artificial intelligence (AI), synthesis prediction has been revolutionized.
AI-driven advances in synthesis prediction have greatly accelerated the drug discovery process. Machine learning algorithms can analyze vast amounts of chemical data and learn patterns and rules that govern successful synthesis. These algorithms can then generate predictions for the most efficient and cost-effective synthetic routes.
Benefits of AI-driven Synthesis Prediction
There are several key benefits to using AI-driven synthesis prediction in drug discovery:
- Time and cost savings: AI algorithms can rapidly analyze large chemical databases and propose synthetic routes, saving both time and money compared to traditional trial-and-error methods.
- Increased success rate: By leveraging the power of AI, chemists can explore a wider range of potential synthetic routes and increase the likelihood of finding a successful one.
- Optimized synthesis: AI algorithms can consider multiple factors, such as reaction conditions, reagent availability, and scalability, to propose the most efficient and practical synthetic routes.
Challenges and Future Directions
While AI-driven synthesis prediction has shown great promise, there are still challenges to overcome. One of the main challenges is the lack of high-quality training data, as experimental data on successful and unsuccessful syntheses is often limited. Additionally, the complex nature of organic chemistry poses difficulties in accurately predicting reaction outcomes.
However, researchers are actively working on addressing these challenges. By collecting and sharing more chemical data, improving machine learning algorithms, and integrating AI with experimental techniques, the field of AI-driven synthesis prediction is expected to continue advancing.
In the future, AI-driven synthesis prediction has the potential to transform the drug discovery process, enabling the rapid and efficient design of new molecules with desired properties. By harnessing the power of AI, scientists can unlock new possibilities in the quest for novel drugs and therapies.
Accelerating Drug Synthesis and Optimization
One of the key challenges in drug discovery is the efficient synthesis of new compounds. Traditional methods of drug synthesis can be time-consuming and expensive, often requiring multiple steps and extensive optimization. However, with the advent of AI in drug discovery, the process of drug synthesis and optimization has been revolutionized.
AI algorithms can analyze vast amounts of data and predict the most efficient synthetic routes for a given compound. By leveraging machine learning techniques, these algorithms can learn from previous successful syntheses and propose optimized routes for new compounds. This not only saves time and resources but also enables the synthesis of complex molecules that were previously considered challenging or even impossible.
Furthermore, AI can also assist in the optimization of drug compounds. By simulating how different modifications to a compound's structure can affect its properties and activity, AI algorithms can suggest modifications that can enhance potency, selectivity, or pharmacokinetic properties. This iterative process of synthesis and optimization can significantly accelerate the drug discovery process and increase the chances of finding successful drug candidates.
Moreover, AI can aid in the design of novel drug candidates by predicting synthetic feasibility. By analyzing the chemical space and the rules governing chemical reactions, AI algorithms can propose virtual compounds that have a high probability of being synthesized successfully. This allows researchers to explore a much larger space of potential drug candidates and increases the chances of discovering novel, effective therapies.
In conclusion, the integration of AI in drug discovery has revolutionized the process of drug synthesis and optimization. By leveraging machine learning and predictive modeling, AI algorithms can accelerate the synthesis process, optimize drug compounds, and even aid in the design of novel drug candidates. This has the potential to greatly impact the pharmaceutical industry, enabling the development of new drugs faster and more efficiently than ever before.
Trial Modeling: AI-powered Innovations
AI-powered trial modeling is revolutionizing the drug discovery process by providing valuable insights into the efficacy and safety of potential drugs. By simulating clinical trials, researchers can obtain crucial information about the drug's effectiveness, potential side effects, and optimal dosing regimens.
One of the key advantages of AI in trial modeling is its ability to analyze massive amounts of data and identify patterns that may not be apparent to human researchers. Machine learning algorithms can sift through vast databases of patient information, genetic data, and medical literature to identify potential drug candidates and predict their performance in clinical trials.
AI-powered trial modeling also helps to optimize the design of clinical trials. By simulating different trial scenarios, researchers can identify the most efficient and cost-effective trial designs. This not only saves time and resources but also increases the likelihood of obtaining accurate and reliable results.
Improved Patient Selection
AI algorithms can analyze patient data to identify specific patient populations that are more likely to respond positively to a drug. This allows researchers to target their trials towards the patients who are most likely to benefit, increasing the chances of success and reducing the risk of adverse events.
Furthermore, AI can help identify potential drug-drug interactions and predict how different patient populations may respond to a combination of drugs. This information is crucial for designing trials that evaluate the safety and efficacy of drug combinations.
Enhanced Safety Assessment
AI-powered trial modeling can predict potential side effects and adverse events based on the drug's molecular properties and its interaction with biological systems. This allows researchers to assess the safety profile of a drug before it enters clinical trials, reducing the risk of unexpected adverse reactions.
Additionally, AI algorithms can analyze real-world data from post-marketing surveillance to identify safety signals that may not have been detected during clinical trials. This helps to ensure that drugs are continuously monitored for potential safety concerns even after they have been approved.
In conclusion, AI-powered trial modeling is revolutionizing the drug discovery process by providing valuable insights into the efficacy and safety of potential drugs. By optimizing trial design, improving patient selection, and enhancing safety assessment, AI is helping researchers bring safer and more effective drugs to market faster.