In recent years, there has been a significant advancement in the field of Artificial Intelligence (AI) and Augmented Reality (AR). These technologies have become increasingly popular and have the potential to enhance virtual experiences in various fields such as gaming, education, healthcare, and...
AI Generation Evaluation - Assessing the Quality of Text, Images, and Video
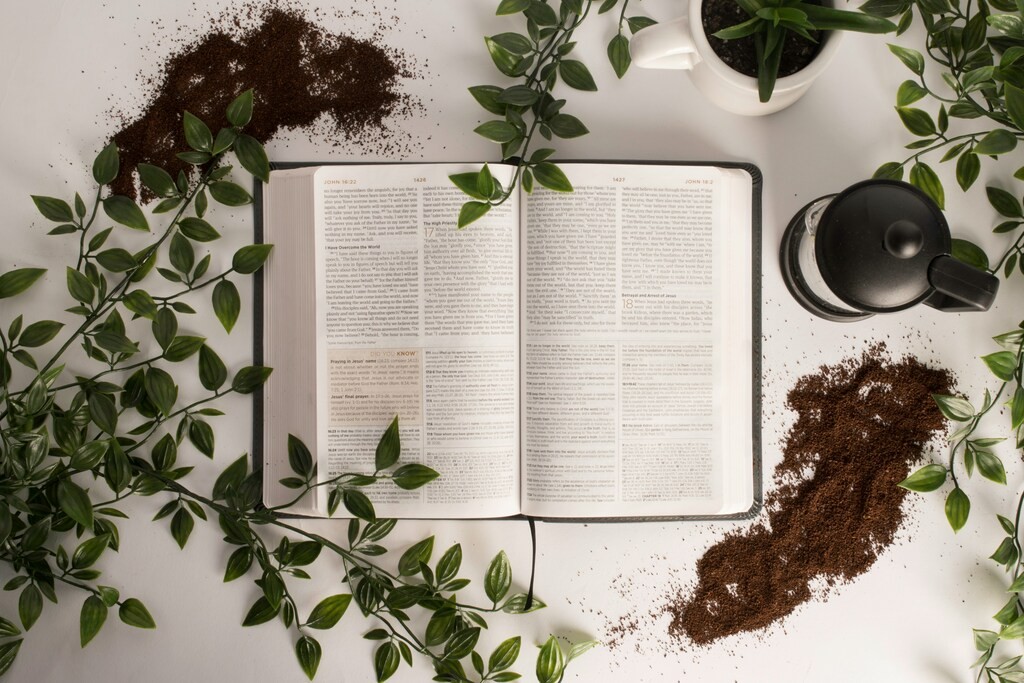
In the ever-evolving world of artificial intelligence (AI), the ability to evaluate the quality of AI-generated content has become paramount. With the rise of AI-powered text, images, and videos, it is crucial to have reliable methods to assess their quality. This article explores the various techniques and approaches used in the evaluation of AI-generated content, focusing on text, images, and videos.
Assessing the quality of AI-generated text involves evaluating its coherence, fluency, and accuracy. Coherence refers to the logical flow of the text, ensuring that the ideas are connected in a meaningful way. Fluency, on the other hand, measures how natural and smooth the text reads. Accuracy is crucial for ensuring that the AI-generated text is factually correct and free from errors. Various evaluation metrics and benchmarks have been developed to assess these aspects of AI-generated text.
When it comes to AI-generated images, evaluating their quality is a multi-faceted task. Factors such as visual appeal, realism, and relevance to the given context need to be considered. Evaluating the visual appeal involves assessing the aesthetics of the image, including its composition, colors, and overall visual impact. Realism refers to how closely the AI-generated image resembles a real photograph or artwork. Relevance, on the other hand, measures how well the image aligns with the given context or prompt. Several evaluation methods, including crowd-sourcing and subjective rating scales, are employed to assess these aspects of AI-generated images.
AI-generated videos present a unique set of challenges in terms of evaluation. In addition to assessing the quality of individual frames, factors such as continuity, storytelling, and overall coherence need to be considered. Evaluating continuity involves ensuring smooth transitions between frames and scenes, while storytelling assesses how well the video conveys a narrative or message. Coherence measures how well the video flows as a whole, ensuring that the sequence of frames is logical and engaging. Various evaluation techniques, such as user studies and expert reviews, are used to assess the quality of AI-generated videos.
In conclusion, the evaluation of AI-generated content, including text, images, and videos, is a crucial task in ensuring its quality and reliability. By employing various evaluation techniques and metrics, researchers and developers can assess the coherence, fluency, accuracy, visual appeal, realism, relevance, continuity, storytelling, and overall coherence of AI-generated content, thus pushing the boundaries of AI technology and enhancing the user experience.
Why AI Generation Evaluation is Important for Assessing Text, Images, and Video Quality
As artificial intelligence (AI) continues to advance and play an increasingly prominent role in our daily lives, the need to evaluate the quality of AI-generated content becomes essential. This evaluation is particularly crucial when assessing text, images, and video, as these mediums heavily influence our perception and understanding of information.
AI generation evaluation allows us to determine the accuracy, coherence, and overall quality of AI-generated content. By assessing the output of AI models, we can identify potential biases, errors, or misleading information that might be present. This evaluation process ensures that the content meets the desired standards and aligns with human expectations.
When it comes to assessing text quality, AI generation evaluation helps us evaluate the grammatical correctness, logical flow, and factual accuracy of AI-generated texts. This is especially important in applications where AI-generated text is used, such as chatbots, news articles, or automated customer support systems. By ensuring the text's quality, we can enhance user experience and avoid spreading misinformation.
Similarly, evaluating the quality of AI-generated images is crucial in various domains, including advertising, design, and entertainment. AI models can generate realistic images, but it is essential to assess whether these images align with the desired objectives and meet the necessary standards. Evaluating image quality helps us identify potential errors, distortions, or inconsistencies, ensuring that the generated images are visually appealing and accurate.
Video evaluation is another critical aspect of AI generation evaluation. With the rise of deepfake technology and AI-generated videos, it becomes crucial to assess the authenticity and credibility of video content. Evaluating video quality allows us to detect manipulations, identify potential deepfakes, and ensure that the content provides an accurate representation of reality. This is necessary to prevent the spread of fake news, misinformation, and deceptive content.
In conclusion, AI generation evaluation plays a vital role in assessing the quality of AI-generated text, images, and videos. It helps us identify biases, errors, and misleading information, ensuring that the content meets the desired standards. By evaluating the accuracy, coherence, and overall quality, we can enhance user experience, prevent the spread of misinformation, and promote the responsible use of AI technology.
The Role of AI in Evaluating Text Quality
Introduction:
- As the digital landscape continues to grow and evolve, the need for accurate and reliable evaluations of text quality becomes increasingly important. With the rise of AI technology, researchers and developers are exploring the role of artificial intelligence in assessing the quality of text.
Challenges in Text Evaluation:
- Evaluating the quality of text is a complex task that requires a deep understanding of language, context, and intent. Human evaluators often rely on their subjective judgments, which can be influenced by biases and personal preferences. Additionally, manual evaluation is time-consuming and costly, making it difficult to scale for large volumes of text.
Benefits of AI in Text Evaluation:
- Artificial intelligence offers several advantages in text evaluation. AI models can analyze text at a large scale, processing vast amounts of information quickly and efficiently. Furthermore, AI algorithms can be trained to recognize patterns and identify linguistic features that contribute to text quality.
Text Quality Metrics:
- AI-based text evaluation systems use various metrics to assess the quality of text. These metrics can include readability, coherence, grammar, and style. By analyzing these factors, AI models can provide objective assessments of text quality, reducing the reliance on subjective human judgments.
Limitations of AI in Text Evaluation:
- While AI has shown great potential in evaluating text quality, there are still limitations to consider. AI models may struggle with understanding nuanced or creative writing styles, as they rely on predefined patterns and rules. Additionally, AI algorithms can be susceptible to biases if the training data is not diverse and representative.
The Future of AI in Text Evaluation:
- As AI technology continues to advance, there is great potential for further improvements in text evaluation. Researchers are working on developing AI models that can better understand context, intent, and cultural nuances. Additionally, efforts are being made to create more diverse and representative training data to reduce biases in AI algorithms.
Conclusion:
- The role of AI in evaluating text quality is becoming increasingly significant. By leveraging AI technology, we can overcome the limitations of manual evaluation and provide more objective and scalable assessments of text quality. While challenges and limitations remain, the future looks promising as AI continues to evolve in this field.
Evaluating Image Quality with AI Generation
As the use of AI technology continues to grow, it is becoming increasingly important to evaluate the quality of images generated by AI algorithms. Evaluating image quality is essential for applications like computer vision, object recognition, and image processing.
One way to assess image quality is through objective metrics. These metrics measure specific aspects of an image, such as sharpness, noise level, color accuracy, and compression artifacts. Objective metrics provide a quantifiable measure of image quality, allowing for easy comparison between different algorithms or image processing techniques.
Another approach to evaluating image quality is through subjective assessment. This involves gathering human feedback on the quality of an image. Human evaluators can rate the overall visual quality, as well as specific aspects like clarity, detail, and aesthetics. Subjective assessment provides a more holistic view of image quality, taking into account factors that may not be captured by objective metrics.
AI algorithms can also be trained to evaluate image quality. By training a model on a dataset of high-quality and low-quality images, the model can learn to classify images based on their perceived quality. This can be done using techniques like convolutional neural networks (CNNs) or generative adversarial networks (GANs). The trained model can then be used to automatically assess the quality of new images.
When evaluating image quality with AI generation, it is important to consider the specific application or use case. Different applications may prioritize different aspects of image quality. For example, a surveillance system may prioritize image clarity and object recognition, while a photography application may prioritize color accuracy and aesthetics.
In conclusion, evaluating image quality with AI generation is a multi-faceted process that involves objective metrics, subjective assessment, and AI-based evaluation. By combining these approaches, developers and researchers can ensure that AI-generated images meet the desired quality standards for various applications.
Assessing Video Quality Using AI Generation Evaluation
Video content has become increasingly prevalent in our digital world, with platforms like YouTube, TikTok, and Netflix driving the demand for high-quality videos. However, assessing the quality of these videos can be a subjective and time-consuming task. That's where AI generation evaluation comes into play.
The Role of AI Generation Evaluation
AI generation evaluation is a powerful tool that leverages artificial intelligence to assess the quality of videos. By training AI models on large datasets of high-quality videos, these models can learn to identify patterns and features that contribute to video quality. This enables them to evaluate the quality of new videos with a high degree of accuracy.
AI generation evaluation takes into account various factors when assessing video quality. These factors include resolution, clarity, color accuracy, frame rate, compression artifacts, and overall visual appeal. By analyzing these factors, AI models can provide objective and consistent evaluations of video quality.
Benefits of AI Generation Evaluation for Video Quality Assessment
Using AI generation evaluation for video quality assessment offers several benefits. Firstly, it significantly reduces the time and effort required to assess video quality manually. Instead of relying on human evaluators, AI models can process and evaluate videos at a much faster rate, allowing for more efficient quality control processes.
Additionally, AI generation evaluation provides more objective and consistent evaluations compared to subjective human evaluations. Human evaluators may have biases or differing opinions on what constitutes good video quality. AI models, on the other hand, follow predefined criteria and algorithms, ensuring a standardized evaluation process.
Furthermore, AI generation evaluation can be scaled to handle large volumes of video content. With the exponential growth of video content on platforms like YouTube and TikTok, manual evaluation becomes impractical. AI models can handle the increased workload and provide evaluations in real-time, enabling faster content moderation and quality assurance.
In conclusion, AI generation evaluation is a valuable tool for assessing video quality. By leveraging artificial intelligence, it offers objective, consistent, and efficient evaluations of video content. As the demand for high-quality videos continues to grow, AI generation evaluation will play a crucial role in maintaining and improving video quality standards.