In recent years, there has been a significant advancement in the field of Artificial Intelligence (AI) and Augmented Reality (AR). These technologies have become increasingly popular and have the potential to enhance virtual experiences in various fields such as gaming, education, healthcare, and...
AI Optimizes Manufacturing Through Predictive Maintenance and Anomaly Detection
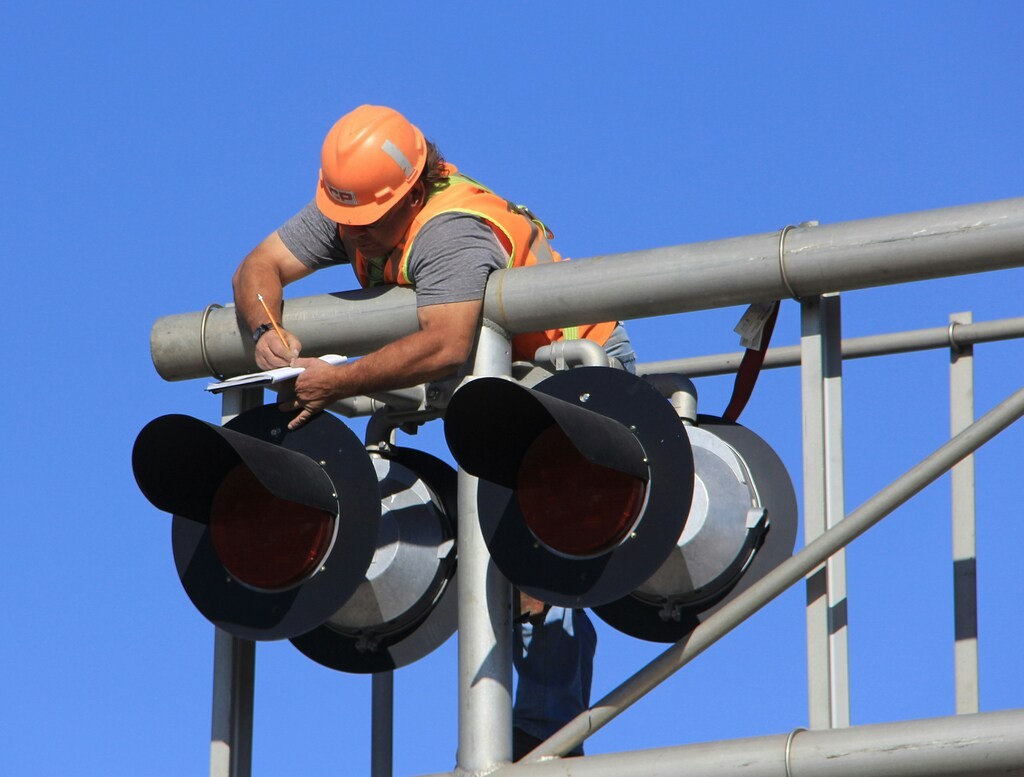
In today's rapidly evolving manufacturing landscape, the ability to optimize production processes is crucial for businesses to stay competitive. One of the key challenges faced by manufacturers is the maintenance of machinery and equipment, which can be costly and time-consuming if not managed efficiently. However, with the advent of artificial intelligence (AI), manufacturers can now leverage predictive maintenance anomaly detection to proactively identify and address potential issues before they escalate.
Predictive maintenance anomaly detection involves the use of AI algorithms and machine learning techniques to analyze real-time data from sensors and other sources. By continuously monitoring the performance of machinery and equipment, AI systems can detect anomalies and deviations from normal operating conditions. This early detection allows manufacturers to take timely corrective actions, preventing unplanned downtime and optimizing the overall efficiency of the production process.
The benefits of AI-powered predictive maintenance anomaly detection are manifold. Firstly, it enables manufacturers to move away from reactive maintenance approaches, where equipment is repaired or replaced only after a breakdown occurs. Instead, manufacturers can adopt a proactive approach, where potential issues are identified and addressed before they lead to costly failures. This not only reduces downtime and maintenance costs but also extends the lifespan of machinery and equipment.
Furthermore, AI algorithms can analyze large volumes of data from various sources, such as historical maintenance records, sensor readings, and operational data. By uncovering patterns and correlations within this data, AI systems can provide valuable insights into the root causes of anomalies and help manufacturers optimize maintenance strategies. This data-driven approach allows manufacturers to prioritize maintenance tasks, allocate resources effectively, and make informed decisions to improve overall equipment effectiveness.
How AI Optimizes Manufacturing
Artificial Intelligence (AI) is revolutionizing the manufacturing industry by optimizing various processes and increasing efficiency. With its predictive capabilities and anomaly detection techniques, AI plays a crucial role in improving manufacturing operations.
Predictive Maintenance
One of the key ways AI optimizes manufacturing is through predictive maintenance. Traditionally, manufacturing equipment is maintained based on a fixed schedule or when a breakdown occurs. This approach is often inefficient and costly. AI enables manufacturers to move from reactive to proactive maintenance by analyzing data from sensors and machinery to predict when maintenance is required.
By using machine learning algorithms, AI can identify patterns and trends in sensor data, allowing manufacturers to predict when equipment failure is likely to occur. This proactive approach helps prevent unplanned downtime, reduces maintenance costs, and increases overall equipment effectiveness.
Anomaly Detection
Another way AI optimizes manufacturing is through anomaly detection. Anomalies in manufacturing processes can lead to defects, quality issues, and costly rework. AI algorithms can analyze large volumes of data in real-time to identify any deviations from normal operating conditions.
By continuously monitoring data from sensors and other sources, AI can quickly detect anomalies and alert operators or trigger automated responses. This early detection allows manufacturers to take corrective actions before defects occur, minimizing waste and improving product quality.
Furthermore, AI can learn from historical data and identify patterns that may indicate potential issues. By analyzing past failures and anomalies, AI systems can predict and prevent similar occurrences in the future, leading to continuous process improvement.
Optimizing Supply Chain
AI can also optimize the manufacturing supply chain by analyzing data from various sources, such as customer demand, inventory levels, and production capacity. By leveraging machine learning algorithms, AI can optimize production schedules, inventory management, and logistics, leading to reduced costs and improved customer satisfaction.
AI can forecast demand more accurately, allowing manufacturers to adjust production levels accordingly and avoid stockouts or excess inventory. It can also optimize routes for transportation, reducing fuel consumption and delivery times.
In conclusion, AI is transforming the manufacturing industry by enabling predictive maintenance, anomaly detection, and supply chain optimization. By harnessing the power of AI, manufacturers can improve efficiency, reduce costs, and enhance overall operations.
Predictive Maintenance for Better Efficiency
Predictive maintenance is a critical component of optimizing manufacturing processes and improving overall efficiency. By leveraging artificial intelligence (AI) and machine learning algorithms, manufacturers can proactively identify and address equipment issues before they lead to costly breakdowns or production delays.
Benefits of Predictive Maintenance
Implementing a predictive maintenance strategy offers several key benefits for manufacturers:
- Reduced downtime: By predicting and preventing equipment failures, manufacturers can minimize unplanned downtime and keep production running smoothly.
- Cost savings: By avoiding costly breakdowns and the associated repair and replacement expenses, manufacturers can save significant amounts of money.
- Improved equipment lifespan: By addressing maintenance issues in a timely manner, manufacturers can extend the lifespan of their equipment and maximize their return on investment.
- Increased safety: Predictive maintenance helps identify potential safety hazards, allowing manufacturers to take proactive measures to protect workers and prevent accidents.
- Better resource allocation: By accurately predicting maintenance needs, manufacturers can allocate resources more efficiently, ensuring that the right personnel and materials are available when and where they are needed.
Implementing Predictive Maintenance
Implementing a predictive maintenance program involves several key steps:
- Data collection: Gather relevant data on equipment performance, including sensor data, maintenance logs, and historical records.
- Data preprocessing: Clean and prepare the collected data for analysis, ensuring that it is accurate and reliable.
- Model development: Train AI and machine learning models on the preprocessed data to detect patterns and anomalies indicative of potential equipment failures.
- Real-time monitoring: Continuously monitor equipment performance in real-time, comparing it to the trained models and generating alerts when anomalies are detected.
- Maintenance planning: Use the insights provided by the predictive maintenance system to plan and schedule maintenance activities, optimizing resource allocation and minimizing production disruptions.
By implementing predictive maintenance, manufacturers can optimize their operations, improve efficiency, and reduce costs, ultimately leading to increased competitiveness in the market.
Anomaly Detection to Prevent Failures
Anomaly detection plays a crucial role in preventing failures in manufacturing processes. By leveraging AI and predictive maintenance technologies, manufacturers can identify deviations from normal operating conditions and take proactive measures to prevent equipment breakdowns.
With anomaly detection, manufacturers can monitor various parameters such as temperature, pressure, vibration, and energy consumption in real-time. By analyzing historical data and identifying patterns, AI algorithms can establish baseline models for normal operation. Any deviations from these models can be flagged as anomalies, signaling potential equipment failures.
By detecting anomalies early on, manufacturers can schedule maintenance interventions before a breakdown occurs. This approach minimizes unplanned downtime, reduces repair costs, and extends the lifespan of equipment.
Furthermore, anomaly detection can also be used to optimize manufacturing processes. By analyzing data from various sensors, AI algorithms can identify inefficiencies and bottlenecks in the production line. Manufacturers can then make data-driven decisions to improve productivity and reduce waste.
In conclusion, anomaly detection is a powerful tool for manufacturers to prevent failures and optimize their manufacturing processes. By leveraging AI and predictive maintenance technologies, manufacturers can ensure smooth operations, reduce downtime, and improve overall efficiency.