In recent years, there has been a significant advancement in the field of Artificial Intelligence (AI) and Augmented Reality (AR). These technologies have become increasingly popular and have the potential to enhance virtual experiences in various fields such as gaming, education, healthcare, and...
AI Revolutionizes Computational Biology — Advancements in Genomics, Imaging, and Modeling
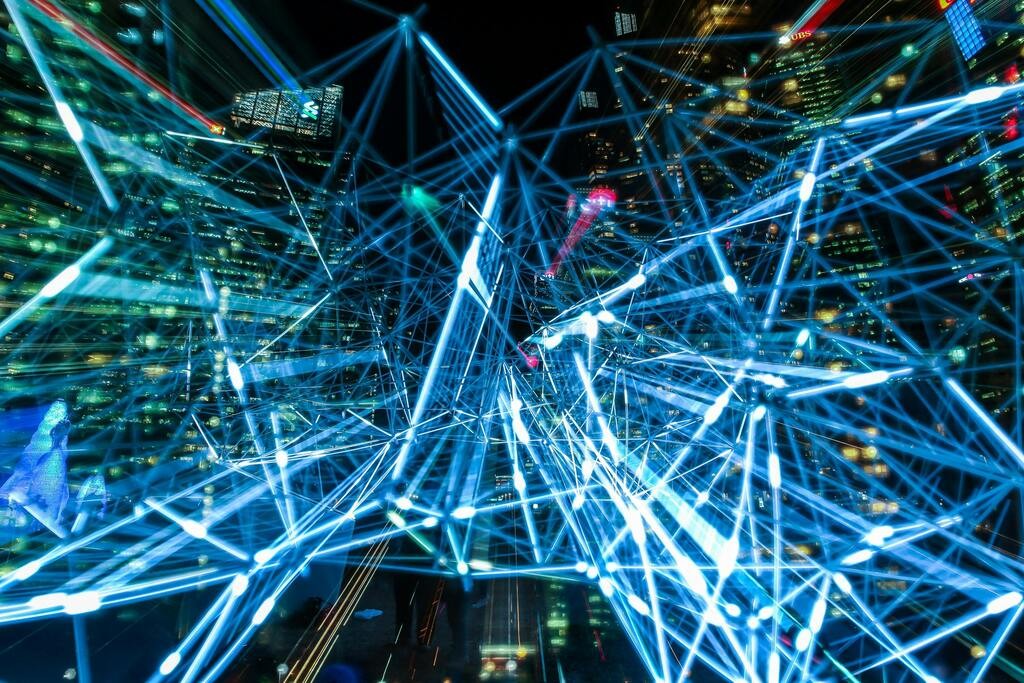
Advances in artificial intelligence (AI) have revolutionized various fields, and one area that has greatly benefited from this technology is computational biology. Computational biology, which combines biology and computer science, aims to solve biological problems using computational techniques. With the advent of AI, researchers in computational biology have been able to make significant advancements in genomics, imaging, and modeling.
Genomics, the study of an organism's complete set of DNA, has seen tremendous progress with the help of AI. Machine learning algorithms have been developed to analyze large-scale genomic data, enabling researchers to identify genetic variations and mutations associated with diseases. This has paved the way for personalized medicine, where treatments can be tailored to an individual's genomic profile, leading to more effective and targeted therapies.
Imaging techniques, such as microscopy and medical imaging, have also been enhanced by AI. AI algorithms can analyze images and identify patterns or anomalies that may be missed by human observers. This has proven invaluable in the field of medical imaging, where early detection of diseases like cancer can significantly improve patient outcomes. Additionally, AI-powered imaging techniques have been instrumental in understanding complex biological processes, such as cell division or protein interactions.
Computational modeling, another area where AI has made significant strides, involves creating computer simulations to study biological systems. AI algorithms can analyze vast amounts of data and generate complex models that simulate the behavior of biological systems, such as protein folding or gene regulation. These models provide valuable insights into the underlying mechanisms of various biological processes, aiding researchers in drug discovery, disease prediction, and understanding fundamental biological principles.
In conclusion, AI has propelled computational biology to new heights, revolutionizing genomics, imaging, and modeling. By harnessing the power of AI, researchers are uncovering new insights into the complexities of the biological world, ultimately leading to improved healthcare, personalized medicine, and a deeper understanding of life itself.
AI Advances in Computational Biology
Computational biology is a rapidly evolving field that combines computer science and biology to solve complex problems in genomics, imaging, and modeling. With the advent of artificial intelligence (AI), there have been significant advances in computational biology, enabling researchers to analyze large-scale biological data and make new discoveries.
Genomics
AI algorithms have revolutionized genomics research by enabling the analysis of vast amounts of genomic data. Machine learning algorithms can identify patterns and relationships in DNA sequences, leading to insights into the function and evolution of genes. AI can also predict the impact of genetic variations on disease risk, helping in the development of personalized medicine.
Furthermore, AI has accelerated the process of genome assembly and annotation, allowing researchers to analyze and understand the entire genetic makeup of an organism. This has led to breakthroughs in understanding the genetic basis of diseases and the development of new treatments.
Imaging
AI has also made significant advancements in the field of biological imaging. Machine learning algorithms can analyze and interpret complex images, such as those obtained from microscopy or medical imaging techniques. This has led to improvements in image segmentation, object recognition, and image classification, enabling researchers to study cellular structures and identify abnormalities.
AI algorithms can also enhance the quality of imaging data by reducing noise and artifacts, improving image resolution, and enabling the visualization of fine details. This has greatly benefited fields such as pathology, where accurate and detailed imaging is crucial for diagnosis and treatment planning.
Advancements in Computational Biology:
- Genomics - Understanding gene function and evolution, personalized medicine
- Imaging - Cellular structure analysis, pathology diagnosis
Overall, AI has revolutionized the field of computational biology, enabling researchers to analyze biological data on a scale and complexity that was not possible before. With further advancements in AI technologies, computational biology is poised to make even greater contributions to our understanding of life and the development of new therapies.
Genomics Imaging Modeling
Genomics imaging modeling is a field that combines genomics, imaging, and computational modeling to gain insights into biological systems at the molecular level. It involves the use of advanced technologies such as next-generation sequencing, microscopy, and machine learning algorithms to analyze and interpret genomic data.
One of the key applications of genomics imaging modeling is in the study of gene expression patterns. By using high-resolution imaging techniques, researchers can visualize the spatial distribution of genes within cells and tissues. This information can then be used to understand how genes are regulated and how their expression patterns change in different biological contexts.
Another important area of genomics imaging modeling is in the analysis of genomic variations. By comparing the genomes of different individuals or populations, researchers can identify genetic variations that are associated with particular traits or diseases. Imaging techniques can be used to validate these findings and provide insights into the functional consequences of these variations.
Computational modeling plays a crucial role in genomics imaging modeling by providing a framework for integrating and analyzing large-scale genomic and imaging data. Models can be used to simulate biological processes, predict the effects of genetic variations, and identify potential drug targets. Machine learning algorithms can also be used to uncover hidden patterns and relationships within complex datasets.
In summary, genomics imaging modeling is a multidisciplinary field that combines genomics, imaging, and computational modeling to study biological systems at the molecular level. It has the potential to revolutionize our understanding of genetics and contribute to the development of personalized medicine.
Applications in Drug Discovery
AI has revolutionized the field of drug discovery by accelerating the process of identifying new drug candidates and optimizing their efficacy. With the help of machine learning algorithms, AI can analyze large datasets and predict the potential of molecules to interact with specific biological targets. This enables researchers to prioritize the most promising compounds for further development.
1. Virtual Screening
Virtual screening is a key application of AI in drug discovery. It involves using computational models to screen large libraries of compounds and identify those with the highest potential for drug activity. AI algorithms can analyze molecular structures and predict their binding affinity to target proteins, helping researchers narrow down the search for potential drug candidates.
2. Drug Repurposing
AI is also being used to identify new uses for existing drugs. By analyzing large databases of drug effects and molecular interactions, AI algorithms can identify potential off-target effects and repurpose drugs for different diseases or conditions. This approach can significantly reduce the time and cost of drug development by leveraging existing knowledge and resources.
Furthermore, AI can help optimize drug dosages and treatment regimens by analyzing patient data and predicting individual responses to different drugs. This personalized medicine approach holds great promise for improving treatment outcomes and reducing adverse effects.
In conclusion, AI has transformed the field of drug discovery by enabling researchers to analyze large datasets, predict molecular interactions, and identify potential drug candidates. The applications of AI in drug discovery are wide-ranging and have the potential to accelerate the development of new treatments and improve patient outcomes.
Challenges and Future Directions
The field of AI in computational biology, genomics, imaging, and modeling is rapidly evolving, with new challenges and opportunities emerging every day. As we continue to push the boundaries of what is possible, it is important to address the key challenges and consider the future directions of this field.
Data Integration and Standardization
One of the major challenges in this field is the integration and standardization of diverse data types. Genomic, imaging, and clinical data are often stored in different formats and come from different sources. Developing efficient methods for combining and analyzing these disparate datasets is crucial for advancing our understanding of complex biological systems. Standardization of data formats and ontologies will also facilitate data sharing and collaboration among researchers.
Interpretability and Explainability
Another important challenge is the interpretability and explainability of AI models in computational biology. Deep learning algorithms, while highly effective, often operate as black boxes, making it difficult to understand the reasoning behind their predictions. Developing methods to interpret and explain AI models will not only improve our trust in their results but also provide valuable insights into biological processes.
Integration of Domain Knowledge
AI models can benefit greatly from incorporating domain-specific knowledge. Integrating existing biological knowledge, such as molecular pathways and gene interactions, into AI models can enhance their performance and interpretability. Developing methods to effectively integrate domain knowledge and AI algorithms will be crucial for the advancement of this field.
Collaboration and Data Sharing
Collaboration and data sharing are essential for the success of AI in computational biology. With the increasing complexity and size of biological datasets, no single research group or institution can tackle all the challenges alone. Establishing collaborative networks and data sharing initiatives will enable researchers from different domains to work together, leverage each other's expertise, and accelerate discoveries in this field.
In conclusion, the field of AI in computational biology, genomics, imaging, and modeling is full of challenges and exciting opportunities. Overcoming these challenges and exploring new directions will not only advance our understanding of biological systems but also pave the way for novel diagnostic and therapeutic approaches in healthcare.