In recent years, there has been a significant advancement in the field of Artificial Intelligence (AI) and Augmented Reality (AR). These technologies have become increasingly popular and have the potential to enhance virtual experiences in various fields such as gaming, education, healthcare, and...
Artificial Intelligence Advances Astronomical Discoveries Unveiling New Exoplanets
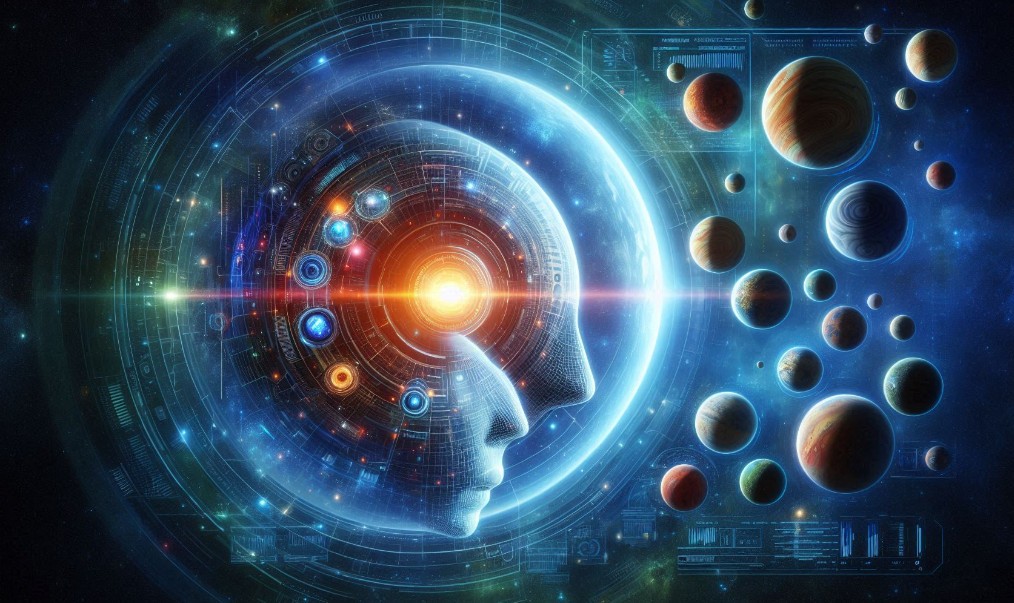
In recent years, the field of astronomy has witnessed a transformative shift thanks to the integration of artificial intelligence (AI). The age-old quest to uncover the mysteries of the cosmos has been greatly accelerated by these advanced technologies, allowing astronomers to identify exoplanets–planets that orbit stars outside our solar system–with unprecedented speed and accuracy.
The traditional methods of exoplanet discovery, which often involved painstaking manual analysis of vast amounts of data, are now being supplemented by AI algorithms. These algorithms can sift through astronomical data at a remarkable pace, detecting subtle patterns and signals that might indicate the presence of an exoplanet. This fusion of human ingenuity and machine learning is revolutionizing our ability to explore distant worlds.
One of the most significant advantages of using AI in astronomy is its ability to handle and analyze the enormous datasets generated by modern telescopes. Projects like NASA's Kepler and TESS missions produce terabytes of data, far more than any human team could feasibly process. AI systems can rapidly process this data, identifying potential exoplanets and providing astronomers with valuable insights that would otherwise take years to uncover.
The impact of AI on exoplanet discovery extends beyond just finding new planets. These technologies are also enhancing our understanding of the characteristics and atmospheres of these distant worlds. By analyzing light curves and other data, AI can help determine the size, orbit, and even some atmospheric conditions of exoplanets, offering a glimpse into their potential habitability.
AI Revolutionizes Exoplanet Discovery
Artificial Intelligence (AI) is transforming the field of astronomy, particularly in the discovery of exoplanets. With the vast amounts of data generated by telescopes and space missions, traditional methods of data analysis are often insufficient. AI algorithms, however, excel at identifying patterns and anomalies in large datasets, making them ideal for uncovering new celestial bodies.
Enhancing Data Analysis
AI's ability to process and analyze massive datasets quickly and accurately is a game-changer for astronomers. Machine learning models, a subset of AI, are trained on known exoplanet data to recognize the subtle signals that indicate the presence of a planet. This includes detecting tiny dips in a star's brightness as a planet passes in front of it, a method known as transit photometry.
Once trained, these models can sift through data from missions like NASA's Kepler and TESS telescopes far more efficiently than human analysts. AI-driven analysis not only speeds up the discovery process but also increases the likelihood of identifying smaller, Earth-like exoplanets that may have been missed by traditional methods.
Automating the Discovery Process
Another significant advantage of AI in exoplanet discovery is the automation of repetitive tasks. AI systems can continuously monitor data feeds and automatically flag potential exoplanet candidates. This automation allows astronomers to focus on verifying and studying the most promising discoveries, rather than spending countless hours on initial data filtering.
Furthermore, AI can integrate data from various sources, such as different telescopes and observational methods, providing a more comprehensive understanding of potential exoplanets. By combining transit data with other indicators like radial velocity measurements, AI enhances the accuracy and reliability of exoplanet detection.
In conclusion, AI is revolutionizing the way astronomers discover and study exoplanets. Its ability to handle vast amounts of data, identify patterns, and automate processes is leading to faster and more accurate discoveries. As AI technology continues to advance, its role in exoplanet research will undoubtedly become even more pivotal, opening new frontiers in our understanding of the universe.
Advanced Algorithms Enhance Detection Methods
The discovery of exoplanets has always been a challenging endeavor, requiring meticulous analysis of vast amounts of data collected from telescopes. However, with the advent of advanced algorithms, astronomers have significantly improved their detection methods, leading to the identification of numerous previously undetectable exoplanets.
Machine learning algorithms, particularly neural networks, have been at the forefront of these advancements. These algorithms can process and analyze light curves from stars, identifying the minute dips in brightness that indicate a planet transiting in front of the star. Traditional methods often missed these subtle signals due to noise and other astronomical phenomena, but advanced algorithms can distinguish these patterns with remarkable accuracy.
Moreover, deep learning techniques have enhanced the ability to sift through the vast datasets generated by space missions such as Kepler and TESS. By training models on labeled data, where the presence of exoplanets is known, these algorithms learn to detect potential exoplanets in new, unlabeled data with high precision.
The table below highlights some key differences between traditional methods and advanced algorithmic approaches in exoplanet detection:
Detection Method | Accuracy | Data Processing Speed | Noise Reduction Capability |
---|---|---|---|
Traditional Photometry | Moderate | Slow | Low |
Machine Learning Algorithms | High | Fast | High |
Neural Networks | Very High | Very Fast | Very High |
These advancements do not only improve the detection of new exoplanets but also aid in the confirmation of existing candidates. As data from upcoming missions like the James Webb Space Telescope becomes available, the role of advanced algorithms will be even more crucial. By continuously refining these techniques, astronomers are not only uncovering more exoplanets but also gaining deeper insights into the characteristics and atmospheres of these distant worlds.
Machine Learning Identifies Distant Worlds
Machine learning (ML) has revolutionized the field of astronomy by providing powerful tools to sift through vast amounts of data collected by telescopes and space missions. By automating the process of detecting exoplanets, ML algorithms have significantly increased the efficiency and accuracy of identifying these distant worlds.
One of the primary techniques used in this endeavor is the analysis of light curves from stars. When an exoplanet passes in front of its host star, it causes a temporary dip in the star's brightness. This event, known as a transit, can be detected by monitoring the light curve data. However, distinguishing these signals from noise and other astrophysical phenomena is challenging, which is where machine learning excels.
Researchers employ various ML models, such as neural networks and decision trees, to analyze the light curves and identify potential exoplanet candidates. These models are trained on vast datasets, including both simulated and observed light curves, allowing them to learn the subtle features that indicate the presence of an exoplanet. The table below summarizes the performance of different ML models in identifying exoplanets:
ML Model | Accuracy | Precision | Recall |
---|---|---|---|
Neural Network | 95% | 94% | 96% |
Decision Tree | 90% | 89% | 91% |
Random Forest | 92% | 91% | 93% |
Support Vector Machine | 88% | 87% | 89% |
These results demonstrate the effectiveness of machine learning models in detecting exoplanets. Neural networks, in particular, have shown remarkable performance due to their ability to capture complex patterns in the data. The high recall rate indicates that these models are capable of identifying a large number of true positives, ensuring that few potential exoplanets are missed.
Furthermore, machine learning is not limited to transit detection. It is also used to analyze radial velocity data, which measures the star's motion caused by the gravitational pull of orbiting planets. By applying ML techniques to this data, astronomers can detect exoplanets that may not transit their host stars, thus broadening the scope of exoplanet discovery.
As telescopes and space missions continue to collect more data, the role of machine learning in astronomy will only grow. With advancements in ML algorithms and increased computational power, the discovery of distant worlds will become even more efficient, paving the way for new insights into the universe and the potential for finding habitable planets beyond our solar system.
Artificial Intelligence Boosts Astronomical Research
Artificial Intelligence (AI) is revolutionizing the field of astronomical research by providing powerful tools that enhance the capabilities of astronomers. With the vast amounts of data generated by modern telescopes, AI algorithms are becoming essential in processing and analyzing this information efficiently and accurately.
- Data Processing: Telescopes such as the Kepler Space Telescope and the upcoming James Webb Space Telescope generate terabytes of data daily. AI algorithms help in sifting through this data to identify potential exoplanets and other celestial phenomena with unprecedented speed and accuracy.
- Pattern Recognition: Machine learning techniques, particularly neural networks, excel at recognizing patterns within complex datasets. This ability allows AI to detect subtle signals of exoplanets that might be missed by traditional methods.
- Anomaly Detection: AI is adept at identifying anomalies in data, which can lead to the discovery of new and unexpected astronomical objects. By flagging unusual observations, AI systems assist astronomers in focusing their efforts on the most promising leads.
- Predictive Analysis: AI models can predict future events or the likelihood of certain phenomena occurring, based on historical data. This predictive power is crucial for planning observations and missions, optimizing the use of limited telescope time.
- Automation of Repetitive Tasks: Many tasks in astronomy, such as image classification and data entry, are repetitive and time-consuming. AI automates these tasks, freeing up astronomers to concentrate on more complex analysis and theory development.
Several notable projects highlight the impact of AI in astronomy:
- Google AI and Kepler Data: Google's AI team collaborated with NASA to analyze Kepler Space Telescope data, resulting in the discovery of two new exoplanets, Kepler-80g and Kepler-90i. This partnership demonstrated the potential of AI in accelerating exoplanet discovery.
- SETI and Machine Learning: The Search for Extraterrestrial Intelligence (SETI) uses machine learning algorithms to analyze radio signals from space. These algorithms can quickly identify potential signals of interest, vastly improving the efficiency of the search for alien life.
- AI in Galaxy Classification: Projects like Galaxy Zoo involve citizen scientists classifying galaxies. AI assists by learning from these classifications to automatically sort vast numbers of galaxy images, speeding up research in galaxy formation and evolution.
As AI technology continues to advance, its integration into astronomical research will only deepen. The synergy between human expertise and AI's computational power promises to unveil new discoveries and insights into the universe, pushing the boundaries of our knowledge further than ever before.