In recent years, there has been a significant advancement in the field of Artificial Intelligence (AI) and Augmented Reality (AR). These technologies have become increasingly popular and have the potential to enhance virtual experiences in various fields such as gaming, education, healthcare, and...
Artificial Intelligence Develops Ability to Anticipate People's Taste Preferences
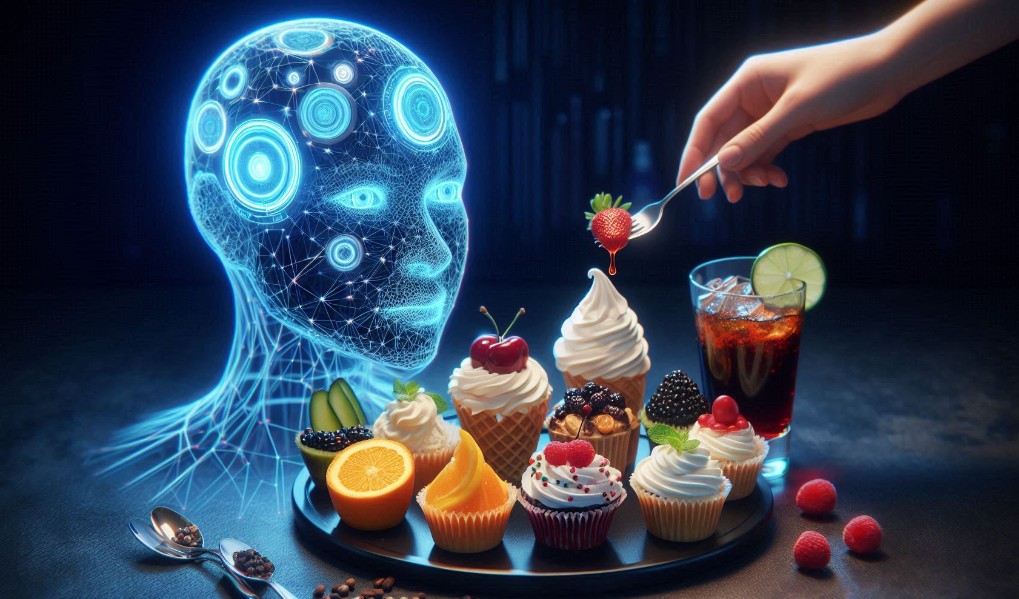
In a world where personalization is becoming increasingly important, artificial intelligence (AI) is revolutionizing the way we understand and cater to individual tastes. From recommending movies and music to suggesting meals and fashion choices, AI systems are being trained to predict what we like with remarkable accuracy. This transformation is not only enhancing user experience but also reshaping industries by providing unprecedented insights into consumer behavior.
At the heart of this technological advancement lies sophisticated algorithms capable of analyzing vast amounts of data. By examining patterns in our past choices and preferences, AI can make educated guesses about what we might enjoy in the future. This predictive power is rooted in machine learning, a subset of AI where systems improve their predictions over time through continuous learning and adaptation. As a result, the more data these systems process, the better they become at understanding and anticipating our desires.
One of the most compelling aspects of AI-driven taste prediction is its potential to offer hyper-personalized experiences. Imagine a world where your streaming service not only suggests movies you might like but also predicts the perfect time for you to watch them based on your schedule and mood. Or consider a shopping platform that curates products tailored specifically to your style and budget. These scenarios are no longer science fiction but are rapidly becoming reality, thanks to the advancements in AI.
However, as AI becomes more adept at predicting our preferences, it also raises important questions about privacy and ethical use of data. Ensuring that these systems are transparent and that users have control over their data is crucial for maintaining trust and fostering a positive relationship between humans and AI. As we continue to explore the capabilities of AI in predicting taste preferences, it is essential to balance innovation with responsible practices.
Artificial Intelligence Revolutionizing Taste Prediction
Artificial intelligence (AI) has made significant strides in understanding and predicting human taste preferences. By leveraging vast amounts of data and sophisticated algorithms, AI systems can analyze patterns and nuances that were previously beyond the grasp of traditional methods. This transformation is not only enhancing personalized recommendations but also revolutionizing various industries such as food and beverage, entertainment, and retail.
One of the key aspects of AI's ability to predict taste preferences lies in its use of machine learning models. These models are trained on diverse datasets that include demographic information, historical preferences, and behavioral data. By continuously learning from new inputs, AI systems become increasingly accurate in their predictions. This dynamic learning process ensures that recommendations remain relevant and personalized.
The impact of AI on taste prediction can be illustrated through the following applications:
Application | Description |
---|---|
Food and Beverage | AI helps in developing new recipes and predicting consumer preferences for flavors, leading to the creation of innovative products tailored to individual tastes. |
Entertainment | Streaming services use AI to recommend movies, TV shows, and music based on users' viewing and listening history, enhancing the overall user experience. |
Retail | Retailers utilize AI to suggest products that customers are more likely to purchase, based on past buying behavior and current trends, thus improving sales and customer satisfaction. |
In the food and beverage industry, AI can analyze taste compounds and predict how different ingredients will interact. This capability enables chefs and food scientists to experiment with new combinations and create dishes that cater to specific palates. Additionally, AI-powered platforms can recommend recipes based on individual dietary restrictions and flavor preferences, making meal planning more convenient and enjoyable.
For the entertainment sector, AI algorithms analyze a user's interaction with content, such as watch time, ratings, and search queries. This information is used to suggest new content that aligns with the user's tastes, thereby increasing engagement and satisfaction. Platforms like Netflix and Spotify have successfully implemented these systems, offering highly personalized content curation.
In retail, AI-driven recommendation engines analyze purchasing data to predict which products customers are likely to buy next. By understanding the preferences and habits of shoppers, retailers can create more targeted marketing campaigns and optimize their inventory. This not only boosts sales but also enhances the shopping experience by making it more tailored to individual needs.
Overall, AI's ability to predict taste preferences is reshaping how businesses interact with consumers. By offering more personalized and relevant recommendations, AI is enhancing customer satisfaction and driving innovation across multiple sectors.
Understanding the Mechanisms of AI Taste Prediction
Artificial intelligence (AI) has made significant strides in understanding and predicting human taste preferences. This capability is built on complex algorithms and models that analyze vast amounts of data to discern patterns and trends. To comprehend how AI predicts taste preferences, it is crucial to delve into the underlying mechanisms that drive this technology.
Several key components contribute to the effectiveness of AI in predicting taste:
- Data Collection: AI systems gather data from a variety of sources, including user interactions, reviews, social media, and purchase histories. This data forms the foundation upon which AI models are built.
- Feature Extraction: From the collected data, AI algorithms identify relevant features that influence taste preferences. These features could be demographic information, past behavior, explicit ratings, and even implicit signals like browsing time or click patterns.
- Machine Learning Models: Advanced machine learning techniques, such as neural networks, decision trees, and collaborative filtering, are employed to analyze the extracted features. These models learn to recognize complex relationships between different factors that influence taste.
- Personalization: AI systems tailor their predictions to individual users by continuously updating their models based on new data. This ensures that the predictions remain relevant and accurate over time.
To better illustrate these mechanisms, consider the following steps that an AI system might take to predict a user's taste in music:
- Data Aggregation: The AI collects data on the user's listening history, including songs played, skipped, and favorited. It may also gather information from social media profiles and music streaming services.
- Pattern Recognition: Using machine learning algorithms, the AI identifies patterns in the user's music preferences, such as favorite genres, artists, and times of day when they listen to music.
- Model Training: The AI trains a model on the collected data, learning to associate certain features with the user's preferences. For example, it might learn that the user prefers upbeat pop music in the morning and calm classical music in the evening.
- Prediction and Adaptation: Based on the trained model, the AI makes predictions about new songs or artists the user might enjoy. It continuously refines its predictions by incorporating feedback from the user's interactions, such as liking or skipping recommended songs.
These mechanisms demonstrate the intricate processes that enable AI to predict human taste preferences accurately. By leveraging sophisticated algorithms and vast datasets, AI systems can provide personalized recommendations that enhance user experiences across various domains, from music and movies to food and fashion.
Applications of AI in the Food Industry
Artificial Intelligence (AI) is revolutionizing the food industry by enhancing various aspects from farm to table. Its applications span across supply chain optimization, food safety, personalized nutrition, and even culinary innovation. By leveraging AI, companies can improve efficiency, reduce waste, and meet consumer demands more accurately.
Supply Chain Optimization
AI algorithms are employed to streamline the food supply chain, ensuring that products are delivered fresh and on time. Predictive analytics can forecast demand, helping suppliers manage inventory more effectively. This reduces overstocking and minimizes waste, contributing to a more sustainable supply chain.
For example, AI can analyze weather patterns, market trends, and historical sales data to predict future demand for specific food items. This enables producers and retailers to adjust their production and stocking strategies accordingly.
Personalized Nutrition
AI is also making strides in personalized nutrition by analyzing individual preferences and health data to recommend tailored meal plans. These systems consider factors such as dietary restrictions, nutrient requirements, and personal taste preferences. AI-driven apps can suggest recipes, monitor dietary intake, and even provide shopping lists to ensure users follow their nutritional goals.
Moreover, AI can track eating habits and provide feedback in real-time, helping users make healthier choices. This personalized approach not only enhances individual health outcomes but also creates a more engaging and satisfying culinary experience.
AI Application | Benefit |
---|---|
Supply Chain Optimization | Reduces waste and improves delivery efficiency |
Personalized Nutrition | Offers tailored meal plans for better health outcomes |
Food Safety Monitoring | Detects contamination and ensures compliance with safety standards |
Product Development | Innovates new recipes and flavors based on consumer data |
In conclusion, AI's integration into the food industry is driving significant advancements. From optimizing supply chains to creating personalized nutrition plans, AI technologies are enhancing efficiency, reducing waste, and providing consumers with customized experiences. As AI continues to evolve, its impact on the food industry will undoubtedly grow, leading to smarter and more sustainable food systems.
Challenges and Future of Predictive AI
Current Challenges
Predictive AI has made significant strides in understanding and anticipating human preferences, but several challenges remain. These challenges hinder the full potential of AI in accurately predicting tastes and preferences. Key challenges include:
- Data Privacy and Security: Collecting and processing vast amounts of personal data raise significant privacy concerns. Ensuring that this data is protected against breaches is critical.
- Bias and Fairness: AI systems can inadvertently learn and perpetuate biases present in the training data. Addressing these biases is essential to provide fair and unbiased predictions.
- Complexity of Human Preferences: Human tastes are highly subjective and can be influenced by numerous factors, including cultural, social, and psychological elements, making accurate prediction challenging.
- Transparency and Explainability: Many AI models, especially deep learning ones, operate as "black boxes," making it difficult to explain how they arrive at specific predictions. This lack of transparency can hinder trust and acceptance among users.
Future Prospects
Despite these challenges, the future of predictive AI holds promising advancements that can significantly enhance its accuracy and applicability. Key future prospects include:
- Improved Data Handling: Advancements in data encryption and anonymization techniques will help address privacy concerns while still allowing AI systems to learn effectively from data.
- Bias Mitigation: Ongoing research into fair AI practices and the development of bias detection and correction algorithms will help create more equitable AI systems.
- Multimodal Learning: Combining data from various sources, such as text, images, and social interactions, can provide a more holistic understanding of user preferences, leading to more accurate predictions.
- Personalization and Adaptability: Future AI systems will be more adaptable, learning continuously from user interactions and feedback to refine and personalize predictions over time.
- Enhanced Explainability: Developing methods for AI explainability will allow users to understand and trust AI predictions better, fostering greater acceptance and integration into daily life.
The integration of these advancements will pave the way for predictive AI to become an integral part of various industries, from entertainment to retail, significantly enhancing user experiences and satisfaction.