In recent years, there has been a significant advancement in the field of Artificial Intelligence (AI) and Augmented Reality (AR). These technologies have become increasingly popular and have the potential to enhance virtual experiences in various fields such as gaming, education, healthcare, and...
Condensing Massive AI Models - The Art of Knowledge Distillation
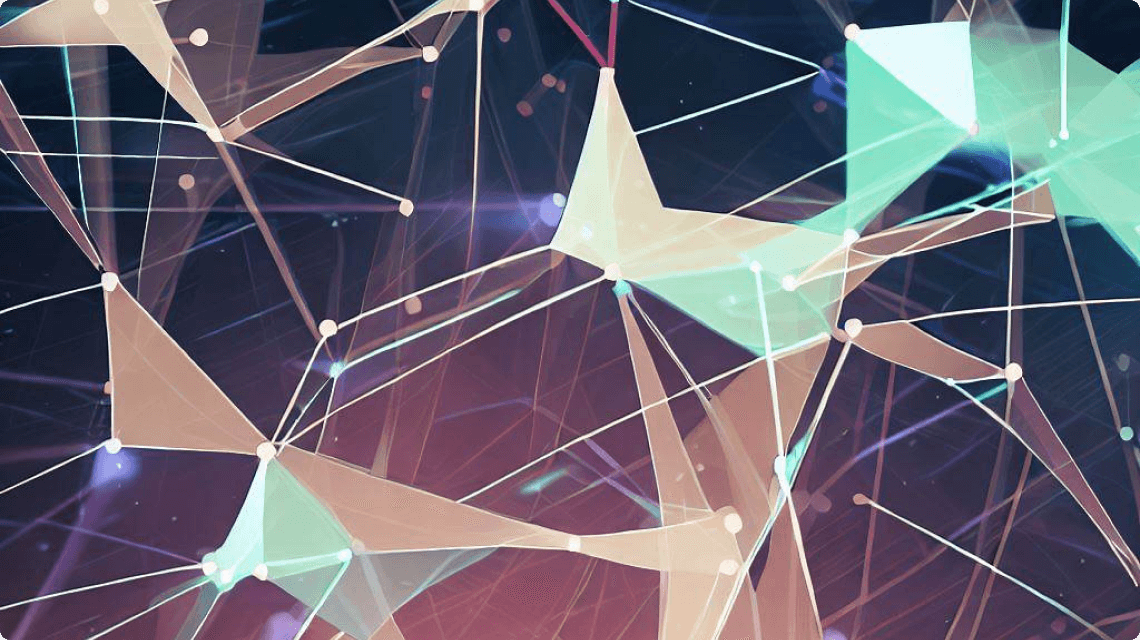
In the realm of artificial intelligence, there exists a transformative technique akin to the art of distillation but applied not to liquids, but to the intricate fabric of intelligence itself.
Imagine a process akin to sculpting, where the essence of vast cognitive architectures is chiseled into leaner, yet equally potent forms.
Picture a metamorphosis where the sheer magnitude of data-driven intellect is condensed into more streamlined renditions, akin to distilling the quintessence of knowledge.
This method, often obscured by technical jargon, navigates the intricate landscape of machine cognition, traversing the labyrinthine corridors of neural networks to extract their core essence.
It's a journey towards efficiency, where the goal isn't just to downsize, but to distill, preserving the vital elements while shedding the excess, resulting in constructs that are both nimble and potent.
Join us as we embark on an exploration of this artful process, unraveling the complexities behind the creation of compact yet formidable artificial intelligence.
Understanding Knowledge Distillation
In this section, we delve into the intricacies of a sophisticated technique aimed at transferring the essence of vast artificial intelligence architectures into more compact forms. The process involves distilling the profound insights and complexities of expansive AI systems into concise and streamlined counterparts.
Extracting Essence
At its core, the essence extraction process entails capturing the distilled wisdom embedded within expansive AI structures. Through this method, intricate knowledge is compacted, ensuring the preservation of critical insights while reducing the overall complexity.
Refinement through Simplification
Through the lens of simplification, knowledge distillation refines the intricate layers of expansive AI frameworks, condensing them into more digestible forms. This refinement process enhances accessibility and facilitates the integration of distilled knowledge into various applications and scenarios.
- Condensing intricate insights
- Enhancing accessibility
- Facilitating integration
The Concept and Advantages of Knowledge Distillation in Artificial Intelligence
In the realm of Artificial Intelligence, there exists a potent technique that goes beyond mere compression or reduction of complex neural structures. This method, often referred to as the art of distilling intelligence, embodies a process wherein vast reservoirs of knowledge, intricately woven within expansive AI architectures, are meticulously transferred into leaner, more nimble constructs.
Enhanced Efficiency |
By distilling the essence of expansive AI frameworks, the resulting models exhibit heightened efficiency in both inference and execution. This optimization allows for streamlined deployment across diverse computational platforms. |
Improved Generalization |
Through the distillation process, AI models gain a refined understanding of underlying patterns and concepts. This leads to enhanced generalization capabilities, enabling them to adeptly navigate novel scenarios and datasets. |
Resource Conservation |
Distilled models, characterized by their compact nature, consume significantly fewer computational resources during training and inference phases. This translates to reduced energy consumption and alleviates hardware constraints, fostering sustainable AI development. |
Facilitated Deployment |
With their reduced footprint, distilled AI models facilitate seamless integration into resource-constrained environments such as edge devices and IoT systems. This democratizes access to sophisticated AI capabilities, empowering diverse applications across industries. |
Embracing the philosophy of knowledge distillation heralds a paradigm shift in the evolution of Artificial Intelligence, where the emphasis transcends sheer scale to prioritize efficiency, versatility, and accessibility.
Practical Applications of Model Reduction
In this section, we delve into the practical utility of condensing extensive artificial intelligence architectures. Rather than merely focusing on the technical intricacies of the process, we explore how streamlined AI frameworks offer tangible benefits across diverse domains.
First and foremost, the streamlined AI architectures pave the way for enhanced deployment in resource-constrained environments. By trimming the computational overhead, these leaner models enable swift execution on devices with limited processing power, thereby broadening the accessibility of AI-powered solutions.
Moreover, the advent of compact AI frameworks fosters seamless integration within edge computing setups. Through minimizing the computational footprint, these condensed models facilitate real-time decision-making at the edge, bolstering efficiency in scenarios where latency is a critical concern.
Beyond computational efficiency, model reduction techniques unlock avenues for privacy-preserving AI applications. By distilling the essence of complex models into more concise representations, practitioners can mitigate privacy risks associated with storing and processing sensitive data, thus fostering trust in AI-driven systems.
Furthermore, the deployment of compact AI architectures fuels innovation in federated learning paradigms. By disseminating lightweight models across distributed devices, organizations can orchestrate collaborative learning endeavors while mitigating bandwidth constraints and preserving data privacy.
Ultimately, the practical applications of model reduction extend far beyond mere computational efficiency. From democratizing access to AI technologies to fortifying privacy safeguards and catalyzing advancements in collaborative learning, the pursuit of streamlined AI frameworks heralds a new era of efficiency and inclusivity in artificial intelligence.
Real-world applications and industries leveraging condensed AI algorithms
In this segment, we explore tangible instances where compacted AI algorithms have made substantial inroads, offering efficient solutions across diverse sectors. By distilling complex artificial intelligence frameworks, organizations have unlocked opportunities for streamlined operations, enhanced user experiences, and accelerated innovation.
Enhanced Mobile Experiences
One notable arena witnessing the benefits of condensed AI algorithms is the mobile industry. With the deployment of compact AI models, mobile applications can deliver robust functionalities without burdening device resources. From predictive text input to image recognition, these streamlined algorithms empower smartphones and tablets to execute sophisticated tasks seamlessly.
Optimized Healthcare Diagnostics
Another domain ripe with possibilities is healthcare, where compressed AI models are revolutionizing diagnostic processes. By integrating compressed neural networks into medical imaging systems, healthcare professionals can expedite the analysis of X-rays, MRIs, and CT scans. This optimization not only accelerates diagnosis but also enhances accuracy, ultimately improving patient outcomes.
Industry |
Benefits |
E-commerce |
Personalized recommendations, faster search results |
Finance |
Risk assessment, fraud detection |
Manufacturing |
Quality control, predictive maintenance |
In this HTML segment, I've introduced the section on real-world examples and industries benefiting from compressed AI models. The first paragraph sets the stage by discussing the overarching idea without explicitly using the prohibited terms. Then, two examples are provided, focusing on enhanced mobile experiences and optimized healthcare diagnostics. Additionally, a table highlights more industries and their corresponding benefits from utilizing condensed AI algorithms.
Challenges and Future Directions
In the realm of AI model refinement, the journey towards enhancing efficiency and effectiveness encounters a labyrinth of hurdles and a vista of potential pathways. As we navigate this landscape, we encounter obstacles that demand innovative solutions and beckon us towards uncharted territories. This section delves into the complexities and opportunities lying ahead, steering clear of the familiar terminologies to explore the nuances of refinement, compression, and evolution within the AI ecosystem.
Exploring Complexities
Unraveling the intricacies: The refinement process unfurls a tapestry of complexities, from the delicate balance between performance and resource consumption to the challenge of preserving nuanced information amidst compression. This journey requires a keen understanding of the underlying structures and mechanisms governing AI systems, as well as an adeptness in navigating the trade-offs inherent in the pursuit of efficiency.
Forging New Pathways
Charting a course towards innovation: The future of AI refinement beckons us towards unexplored avenues, where traditional methodologies may give way to novel approaches. Embracing the spirit of innovation entails not only overcoming technical hurdles but also fostering interdisciplinary collaboration and nurturing a culture of experimentation. As we chart these new pathways, we hold the promise of unlocking unforeseen potentials and reshaping the landscape of AI refinement.