In recent years, there has been a significant advancement in the field of Artificial Intelligence (AI) and Augmented Reality (AR). These technologies have become increasingly popular and have the potential to enhance virtual experiences in various fields such as gaming, education, healthcare, and...
Deciphering Rewards - Unveiling Reward Functions through Inverse Reinforcement Learning from Demonstrations
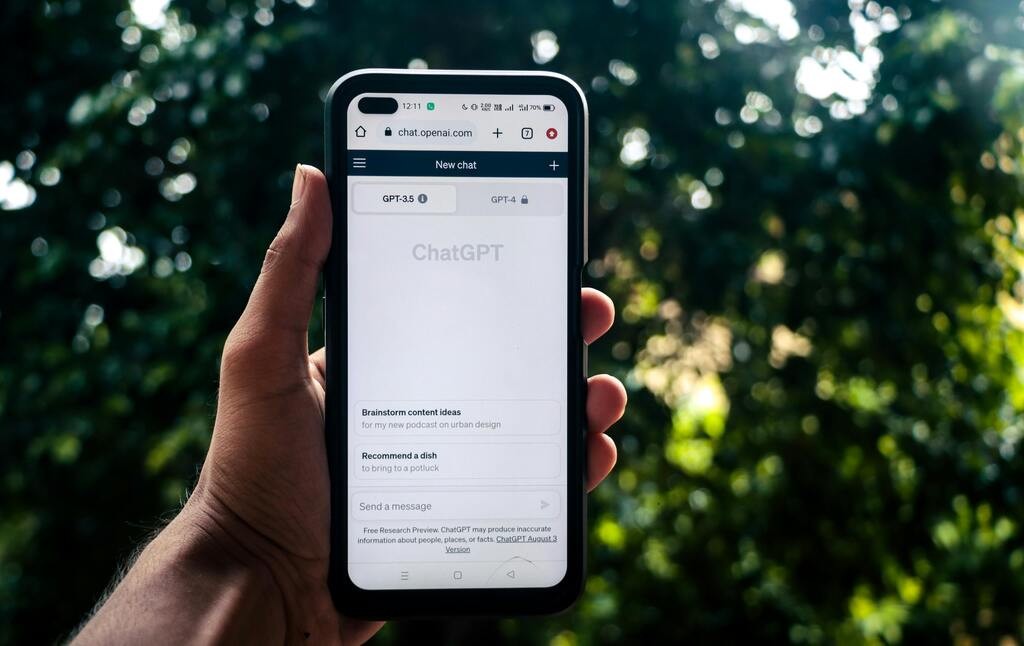
Within the realm of computational intelligence lies a fascinating pursuit, a quest to decode the subtle cues embedded within human actions. This endeavor revolves around discerning the intrinsic motivations steering our behaviors, unraveling the intricate tapestry of intentions woven into our every move.
Inverse inference, a cornerstone of this cognitive odyssey, serves as the conduit through which machines learn to perceive the latent desires guiding human endeavors. Through a delicate dance of observation and interpretation, these algorithms endeavor to glean the underlying rationale behindnetwork error
Inverse Reinforcement Learning: Unraveling the Essence of Reward Inference
In this segment, we delve into the fundamental essence of deciphering the intrinsic motivations guiding agent behavior through a process akin to uncovering hidden treasures within a labyrinth. Rather than directly imparting knowledge, we embark on a quest to decode the intricate signals embedded within the actions of proficient agents.
Navigating the Terrain of Motivational Inference
Embarking on our journey, we navigate through the intricate terrain of motivational inference, where the subtle nuances of human behavior become our guiding stars. Through a process akin to reverse-engineering, we unravel the enigmatic threads of intentionality woven into the fabric of observed actions.
Exploration |
Discovery |
Insight |
Traversing diverse scenarios |
Unveiling hidden motives |
Gaining profound understanding |
Deciphering behavioral patterns |
Exposing latent preferences |
Interpreting underlying incentives |
Interrogating agent decisions |
Eliciting tacit knowledge |
Discerning implicit rewards |
Through this meticulous exploration, we strive to bridge the chasm between observed behavior and the latent motivations steering the course of action, thus shedding light on the intricate dynamics of reward inference.
Theoretical Underpinnings and Core Notions
Exploring the foundational principles and fundamental concepts in this realm delves into the bedrock of understanding human behavior and decision-making processes. This section elucidates the theoretical framework that underpins the extraction of guiding principles from observed behaviors, without explicitly relying on conventional terms often associated with this field.
Underlying Philosophies
At its core, this discipline grapples with deciphering the implicit motivations and underlying rationales embedded within human actions. By elucidating the latent drivers steering observable behaviors, we endeavor to uncover the intrinsic fabric of decision-making dynamics.
Key Abstract Constructs
Within this domain, abstract constructs serve as the cornerstone for elucidating intricate behavioral patterns. These conceptual frameworks offer a lens through which to interpret and analyze observed demonstrations, shedding light on the subtle nuances of human decision-making without recourse to explicit reward functions or traditional learning paradigms.
Applications in Autonomous Systems and Robotics
In the realm of independent systems and mechanical engineering, the utilization of sophisticated methodologies to comprehend and interpret human-guided demonstrations has surfaced as a pivotal advancement. This section delves into the practical implications of such methodologies within the domain of self-governing mechanisms and robotic systems. Here, we explore the integration of intricate algorithms to discern and derive actionable insights from human-provided examples, fostering autonomy and adaptability in mechanical constructs.
Enhanced Autonomy: Through the amalgamation of cognitive frameworks and computational models, autonomous systems stand to achieve unprecedented levels of self-reliance and decision-making capabilities. By assimilating nuanced behavioral cues from human interactions, these systems can refine their operational strategies, thereby navigating intricate environments with heightened precision and efficiency.
Robotic Adaptability: The infusion of interpretive algorithms enables robots to dynamically adjust their actions and responses in accordance with the demonstrated behaviors of human counterparts. This adaptability fosters seamless collaboration between humans and machines, facilitating fluid interaction in diverse scenarios ranging from industrial settings to everyday household chores.
Efficient Task Execution: Leveraging insights gleaned from human demonstrations, robotic systems can optimize their task execution processes, streamlining operations and mitigating inefficiencies. By discerning implicit preferences and priorities embedded within human actions, these systems can tailor their approaches to task completion, augmenting productivity and resource utilization.
Real-world Deployment: The practical implications of inferring actionable knowledge from human demonstrations extend beyond theoretical frameworks, manifesting in tangible applications across various sectors. From autonomous vehicles navigating complex traffic scenarios to robotic assistants aiding in healthcare settings, the integration of interpretive methodologies catalyzes advancements in real-world deployment, shaping the landscape of autonomous systems and robotics.
In summary, the integration of interpretive methodologies within autonomous systems and robotics heralds a paradigm shift, fostering enhanced autonomy, adaptability, and efficiency. By discerning and deriving actionable insights from human-guided demonstrations, these systems transcend traditional boundaries, navigating the complexities of real-world environments with unprecedented sophistication and efficacy.
Ethical Considerations and Paths Forward
In exploring the realm of understanding human behavior through observed actions, there emerge profound ethical considerations and promising trajectories for future inquiry. This section delves into the ethical implications inherent in deciphering the motivating factors behind human actions, and outlines potential avenues for further exploration and refinement.
Navigating Ethical Quandaries
Delving into the intricacies of human behavior through observed demonstrations entails grappling with a spectrum of ethical dilemmas. Understanding the underlying motivations behind actions may unearth sensitive information about individuals, raising concerns regarding privacy, consent, and autonomy. Moreover, the potential for biases to seep into inferred insights poses a significant ethical challenge, highlighting the importance of transparency and accountability in algorithmic decision-making processes.
Charting Future Trajectories
Despite the ethical complexities, the pursuit of deciphering reward structures from demonstrations offers promising avenues for both academic inquiry and real-world application. By fostering interdisciplinary collaboration between computer science, psychology, and ethics, researchers can develop robust frameworks that prioritize ethical considerations while advancing our understanding of human behavior. Moreover, initiatives aimed at promoting diversity and inclusivity in dataset collection and algorithmic design can mitigate the risk of perpetuating biases, paving the way for more equitable and socially responsible applications of inverse reinforcement learning.