In recent years, there has been a significant advancement in the field of Artificial Intelligence (AI) and Augmented Reality (AR). These technologies have become increasingly popular and have the potential to enhance virtual experiences in various fields such as gaming, education, healthcare, and...
Demystifying AI - Ensuring Transparency in Machine Learning for Accountability
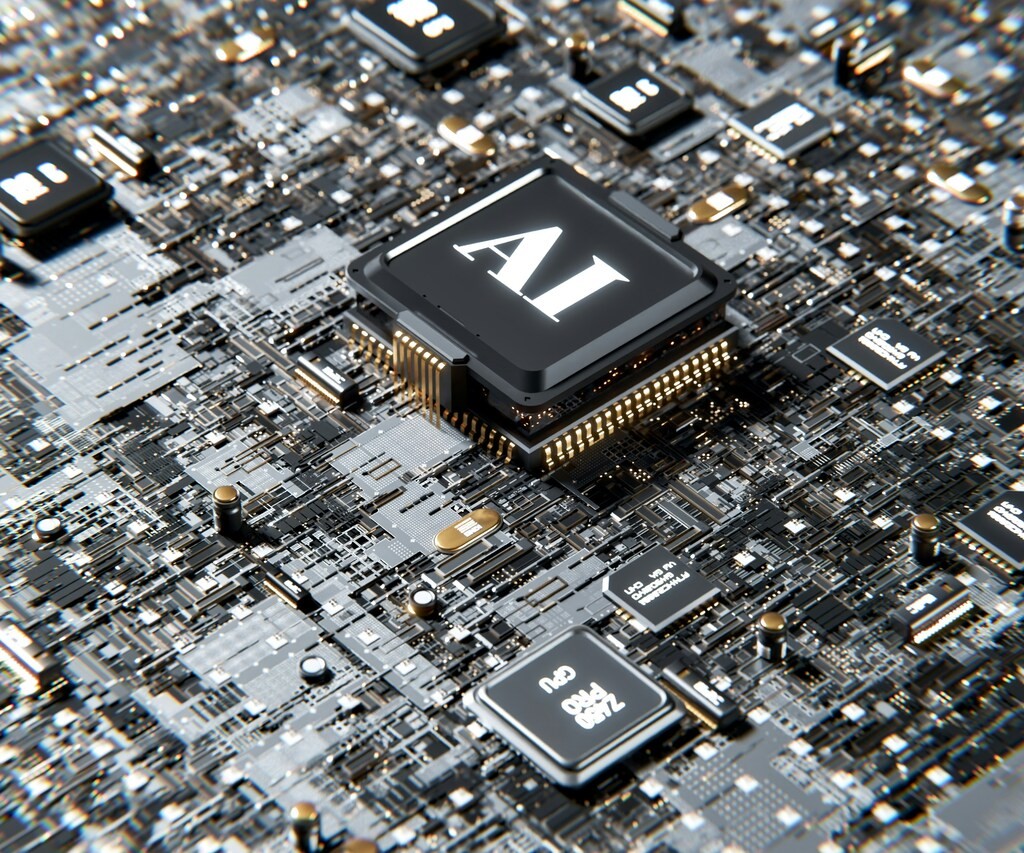
Understanding the Enigma: In the realm of modern technological advancements, the burgeoning significance of elucidating the inner workings of artificial intelligence systems has become an imperative pursuit. Delving into the labyrinthine complexity of computational algorithms, we embark on a journey to unravel the enigmatic nature of AI processes.
Unveiling the Veil: Within this intricate tapestry of digital intelligence, the necessity to shed light on the opacity that shrouds algorithmic decision-making emerges as a pivotal endeavor. The quest for transparency transcends mere scrutiny; it embodies the quest for clarity, fostering comprehension in the face of algorithmic mystique.
Embarking on a Quest: As we navigate the landscape of AI accountability, we are compelled to embark on a quest for discernment, a voyage that transcends the surface-level comprehension of AI functionalities. Beyond the binary confines of inputs and outputs lies the essence of accountability, beckoning us to explore the depths of algorithmic rationale.
Understanding Explainable AI
In this section, we delve into the comprehension of AI systems that unveil their inner workings and reasoning. Instead of cloaking their operations in mystery, these systems shed light on their decision-making processes, fostering transparency and comprehension.
Transparency in Artificial Intelligence
At the core of understanding Explainable AI lies the concept of transparency. It entails the unveiling of the intricate mechanisms and algorithms employed by AI systems to reach conclusions. Transparency serves as a window into the black box of AI, allowing stakeholders to grasp the rationale behind decisions without delving into technical intricacies.
Fostering Trust and Comprehension
Explainable AI endeavors to foster trust and comprehension among users and stakeholders. By elucidating the rationale behind AI-driven decisions, it empowers individuals to comprehend, critique, and augment the systems they interact with. This comprehension not only enhances accountability but also facilitates collaboration between humans and machines, leading to more informed and ethical outcomes.
- Demystifying AI decision-making processes
- Enhancing transparency and comprehension
- Fostering trust and collaboration
Exploring the Concept and Importance
In this section, we delve into the essence and significance of enhancing transparency and comprehensibility within the realm of artificial intelligence systems. The overarching aim is to shed light on the fundamental principles driving the need for clarity and the pivotal role it plays in fostering trust and reliability.
- Understanding the Essence: Here, we embark on a journey to unravel the core principles underpinning the notion of elucidating AI processes. We dissect the intricacies of transparency and elucidate its multifaceted nature, encompassing aspects of clarity, intelligibility, and explicability.
- Significance in Accountability: We then pivot to the pivotal role of transparency in ensuring accountability within AI frameworks. We elucidate how fostering transparency fosters accountability by allowing stakeholders to comprehend the rationale behind AI decisions, thereby enabling them to assess and mitigate potential biases or errors.
- Building Trust and Reliability: Next, we explore how transparency serves as a cornerstone for building trust and reliability in AI systems. By demystifying the inner workings of algorithms and models, stakeholders can develop confidence in AI-driven outcomes, fostering acceptance and adoption.
- Empowering Stakeholders: Lastly, we discuss the empowerment that comes with transparent AI systems. Through comprehensible explanations and interpretable outputs, stakeholders gain agency to validate, challenge, and refine AI processes, ultimately contributing to a more equitable and accountable technological landscape.
Methods for Deciphering AI Decisions
In this section, we delve into various approaches aimed at unraveling the decisions made by artificial intelligence systems. Understanding the rationale behind AI decisions is paramount in ensuring transparency and fostering trust in these automated systems. Through employing diverse techniques, we endeavor to shed light on the inner workings of AI algorithms, enabling stakeholders to comprehend the thought processes driving their outputs.
Feature Importance Analysis
One method involves conducting feature importance analysis, which entails identifying the variables or attributes most influential in determining AI outcomes. By scrutinizing the significance of each feature, stakeholders can discern which factors carry the greatest weight in the decision-making process. Techniques such as permutation importance, SHAP (SHapley Additive exPlanations), and LIME (Local Interpretable Model-agnostic Explanations) offer insights into the relative contributions of different features, facilitating a clearer understanding of AI decisions.
Decision Rule Extraction
Another approach revolves around extracting decision rules from AI models, thereby elucidating the logic guiding their predictions or classifications. By distilling complex algorithms into comprehensible rulesets, stakeholders can gain actionable insights into how input data translates into output decisions. Methods such as decision tree induction, rule-based learning, and symbolic regression aim to uncover the underlying decision-making principles embedded within AI systems, empowering users to interpret and validate their outputs.
Method |
Advantages |
Disadvantages |
Feature Importance Analysis |
Provides insight into influential factors Applicable across various AI models |
May oversimplify complex interactions Requires careful consideration of feature relevance |
Decision Rule Extraction |
Offers transparent decision logic Facilitates human-understandable explanations |
May struggle with capturing nuanced relationships Prone to overfitting with complex datasets |
Techniques and Tools Enhancing Model Transparency
In this segment, we delve into various methodologies and instruments aimed at augmenting the clarity and comprehensibility of AI algorithms. By elucidating the inner workings of these systems, we endeavor to foster a deeper understanding of their functionality and decisions.
Algorithmic Techniques
Algorithmic methodologies play a pivotal role in enhancing model transparency. Through techniques such as feature importance analysis, saliency mapping, and surrogate modeling, practitioners can gain insights into the factors driving model predictions. These methods illuminate the decision-making process, shedding light on the intricate interplay of variables within the algorithm.
Visualization Tools
Visualization tools serve as indispensable aids in elucidating complex AI models. Heatmaps, decision trees, and partial dependence plots are just a few examples of visualization techniques that facilitate the interpretation of machine learning outcomes. By rendering abstract concepts into intuitive graphical representations, these tools empower stakeholders to discern patterns, trends, and anomalies within the data, thereby fostering trust and accountability.
Technique |
Advantages |
Limitations |
Feature Importance Analysis |
Reveals crucial variables influencing predictions |
May overlook complex interactions between features |
Visualization Tools |
Facilitates intuitive interpretation of model behavior |
Depends on the quality of visualizations and human interpretation |
Surrogate Modeling |
Provides simplified approximation of complex models |
May introduce biases inherent to the surrogate model |
Applications of Understandable AI in Real-world Scenarios
In this section, we explore the practical applications of comprehensible AI in various real-world settings. By delving into concrete examples, we illustrate how transparent AI systems play a pivotal role in enhancing decision-making processes across diverse domains.
Industry |
Application |
Benefit |
Healthcare |
Diagnostic Assistance |
Empowering healthcare professionals with insights into AI-generated diagnoses, aiding in accurate and timely patient treatment. |
Finance |
Credit Scoring |
Providing transparency in credit scoring algorithms, enabling individuals to understand factors influencing their creditworthiness. |
Legal |
Evidence Assessment |
Assisting legal practitioners in comprehending AI-generated recommendations for evidence assessment, ensuring fair and just legal proceedings. |
Manufacturing |
Quality Control |
Facilitating understanding of AI-driven quality control processes, enhancing product reliability and customer satisfaction. |
Transportation |
Route Optimization |
Offering insights into AI-based route optimization algorithms, optimizing travel times and reducing environmental impact. |
These examples underscore the significance of transparent AI systems in fostering trust, improving decision-making, and ensuring accountability across a spectrum of real-world scenarios.