In recent years, there has been a significant advancement in the field of Artificial Intelligence (AI) and Augmented Reality (AR). These technologies have become increasingly popular and have the potential to enhance virtual experiences in various fields such as gaming, education, healthcare, and...
Exploring Deeper Dimensions of AI - Unraveling Causal Reasoning Beyond Statistical Associations
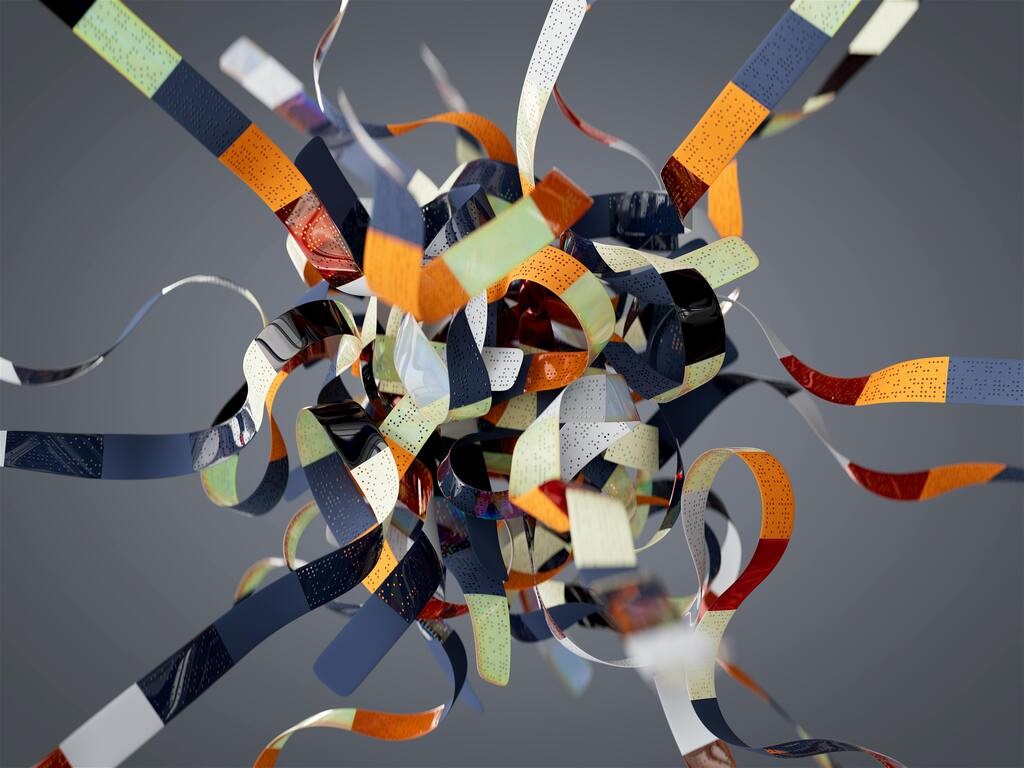
In the realm of artificial intelligence exploration, lies a profound quest not merely for patterns and associations, but for the very essence of causation itself. In the vast labyrinth of data analysis and machine learning, lies an intriguing pursuit to decipher the underlying mechanisms that propel events, actions, and outcomes. It transcends the mere surface of statistical inference, delving deep into the intricate web of cause and effect, seeking to unveil the hidden connections that shape our understanding of the world.
Within the corridors of AI research, lies a shift towards embracing not just the observable relationships, but the fundamental forces that govern them. It's a journey of discovery, where algorithms cease to be content with mere predictions based on correlations, but aspire to comprehend the very fabric of causality that threads through our universe. It's a paradigmatic shift, from the passive observer to the active participant in unraveling the mysteries of causation.
This article embarks on an odyssey through the evolving landscape of AI, where the pursuit of causality transforms from a philosophical pondering to a tangible endeavor. It examines the pivotal role of causal reasoning in reshaping how machines learn, adapt, and ultimately, comprehend the intricate dance of cause and effect in our complex reality. Join us as we delve into the heart of AI, where the quest for understanding transcends statistical confines, leading us towards a deeper comprehension of the world we seek to emulate.
Understanding Cause and Effect in AI Systems
Delving into the essence of AI’s comprehension of causality unveils a profound journey. It's not merely about discerning patterns or deducing outcomes from data points. Rather, it involves deciphering the intricate web of relationships between events, grasping the fundamental principles that drive changes, and discerning the true agents behind observed effects.
Unraveling the Threads of Influence: Within the realm of AI, understanding causality necessitates a departure from the surface-level associations prevalent in statistical analyses. It entails probing deeper into the underlying mechanisms that connect variables and uncovering the directionality of influence.
Perceiving the Dynamics: AI systems must transcend mere observation of co-occurrences to comprehend the dynamics of cause and effect. This entails recognizing how interventions in one aspect can ripple through the system, elucidating the dynamics that govern change.
Inferring Intent and Agency: Beyond statistical correlations, AI must discern the intentions and agency embedded within causal relationships. It involves recognizing not just what happens, but why it happens and who or what instigates the change.
Embracing Complexity: Causal reasoning in AI demands an embrace of complexity, acknowledging the multifaceted interplay of variables and the nonlinear nature of causal pathways. It's about navigating through uncertainty and emergent properties to uncover the true drivers of change.
Exploring the Foundation of Logical Deduction
Within the realm of artificial intelligence, there exists a fundamental quest to decipher the essence of logical deduction, to unravel the intricate threads that weave through causality and inference. This journey delves into the very bedrock of understanding, seeking to illuminate the underlying principles that govern the relationships between events and their consequences.
Unveiling the Architecture of Causal Inference
At the heart of our exploration lies the pursuit of unraveling the structural blueprint of causal inference, the intricate network of logical pathways that guide our understanding of cause and effect. Through meticulous analysis and scrutiny, we aim to decipher the mechanisms that underpin the process of discerning causal relationships from mere correlations.
Charting the Terrain of Logical Induction
Beyond the surface level of statistical associations, lies the terrain of logical induction, where inference transcends the realm of probability to unveil the true nature of causality. Through the lens of deductive reasoning and logical coherence, we navigate this complex landscape, forging a path towards a deeper comprehension of the causal fabric that envelops our world.
Aspect |
Exploration |
Foundational Principles |
Delving into the bedrock of logical deduction |
Inferential Structures |
Unveiling the architecture of causal inference |
Logical Induction |
Charting the terrain beyond statistical correlations |
Unlocking the Potential of AI through Exploring Causality
In this section, we delve into the untapped capabilities residing within the realm of causal inference, traversing beyond mere statistical associations. By embarking on this journey, we aim to unearth the profound impact of causal understanding on the landscape of artificial intelligence. Rather than merely navigating through surface-level correlations, we aim to illuminate the pathways that lead to deeper comprehension and more informed decision-making within AI systems.
Applications Across Diverse Sectors
In this section, we explore the practical implementations of discerning causality beyond mere statistical associations across a spectrum of industries. Delving into realms ranging from healthcare to finance, we unveil how unraveling causal relationships fosters innovation, enhances decision-making processes, and augments efficiency across various sectors.
Challenges and Future Directions in Explicating Causal Insights
In exploring the frontier of unraveling cause-and-effect dynamics within AI systems, myriad hurdles stand as formidable barriers while simultaneously pointing towards promising pathways for advancement. This section delves into the intricacies of these challenges and envisages potential trajectories for the evolution of causal inference in artificial intelligence.
1. Semantic Ambiguity and Contextual Complexity
One of the foremost challenges lies in navigating the labyrinth of semantic ambiguity inherent in causal relationships, compounded by the contextual complexity of real-world data. Unraveling the intricate tapestry of causation demands not only discerning direct causal links but also accounting for indirect, latent influences that often evade simplistic statistical scrutiny.
2. Bridging the Gap between Correlation and Causation
The perennial quest to bridge the chasm between correlation and causation remains a focal point of contention and innovation. While statistical correlations furnish invaluable insights, the leap to establishing causal relationships necessitates nuanced methodologies capable of discerning genuine causal mechanisms from mere coincidental associations.
- Addressing the 'Post Hoc Ergo Propter Hoc' Fallacy
- Developing Robust Counterfactual Reasoning Frameworks
- Enhancing Explainability and Interpretability
These endeavors pave the way towards elucidating causal pathways with heightened fidelity and confidence, fostering the maturation of AI systems endowed with causal reasoning capabilities.