In recent years, there has been a significant advancement in the field of Artificial Intelligence (AI) and Augmented Reality (AR). These technologies have become increasingly popular and have the potential to enhance virtual experiences in various fields such as gaming, education, healthcare, and...
Preserving Privacy Through Collaborative AI Model Training with Federated Learning
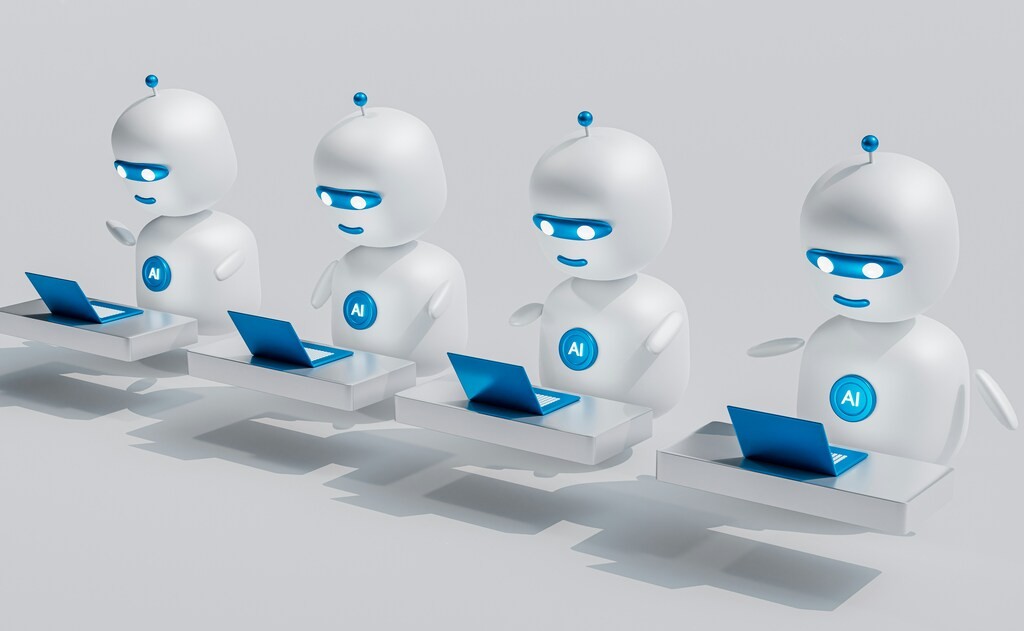
In the realm of artificial intelligence, innovation continuously pushes the boundaries of what is possible. One such advancement revolves around the synergistic orchestration of multiple entities to refine algorithms without compromising individual data security.
This transformative methodology operates on the principle of collective intelligence, harnessing the strengths of diverse sources while safeguarding the sanctity of personal information.
By intertwining the prowess of distributed networks with cutting-edge encryption techniques, this paradigm shift ensures that insights are gleaned collaboratively, yet identities remain obscured behind layers of digital armor.
Federated Learning: Revolutionizing AI Collaboration
In the realm of artificial intelligence cooperation, a groundbreaking paradigm is emerging, reshaping the landscape of collaborative intelligence augmentation. This innovative approach heralds a transformative shift in the way AI systems engage in collective knowledge enhancement without compromising individual data sovereignty or divulging sensitive information. By harnessing the power of distributed networks and decentralized computation, this novel methodology fosters synergistic advancement while safeguarding the sanctity of personal privacy.
Redefining Conventional Wisdom: Gone are the days of centralized model refinement, where data monopolies reigned supreme, extracting insights at the expense of user confidentiality. Instead, a new dawn emerges, characterized by distributed consensus-building mechanisms that empower each participant to contribute meaningfully without forfeiting control over their proprietary information. This departure from traditional paradigms not only democratizes the AI landscape but also cultivates a culture of inclusivity and trust among stakeholders.
Empowering Individual Autonomy: At the heart of this revolution lies a profound commitment to individual sovereignty and data integrity. By encapsulating learning processes within localized environments, each entity retains jurisdiction over its proprietary data assets, while still benefiting from the collective wisdom amassed through federated collaboration. This empowerment not only engenders a sense of ownership but also fosters a virtuous cycle of innovation, where contributions from diverse sources enrich the collective intelligence without compromising individual autonomy.
Forging New Frontiers: As this paradigm gains momentum, it opens doors to unprecedented possibilities in AI cooperation. From cross-domain knowledge synthesis to real-time adaptation, federated learning transcends conventional boundaries, paving the way for novel applications across diverse domains. By embracing this collaborative ethos, stakeholders stand poised to unlock the full potential of distributed intelligence, ushering in a new era of innovation and discovery.
Unlocking the Power of Decentralized Model Training
In the realm of collaborative artificial intelligence advancement, lies a transformative approach poised to revolutionize the way algorithms are honed: decentralized model training. This innovative methodology epitomizes a paradigm shift in the landscape of algorithmic enhancement, embodying principles of distributed intelligence orchestration and privacy-conscious collaborative refinement.
Decentralization Amplified: Embracing a decentralized ethos, this approach fosters a networked ecosystem where algorithmic refinement transcends the confines of centralized control. By leveraging distributed nodes, each endowed with computational prowess, the collective intelligence converges towards a harmonized model, enriched by diverse perspectives and datasets. |
Empowering Privacy: Embedded within the fabric of decentralized model training is a commitment to privacy preservation. Through a mosaic of encryption techniques and anonymized data exchanges, individual data sovereignty is upheld without compromising the collective pursuit of algorithmic excellence. This equilibrium between privacy and progress heralds a new era of responsible AI innovation. |
Synergy in Diversity: Decentralized model training embraces the heterogeneity of data sources and computational infrastructures, transcending the limitations of homogenous datasets and hardware specifications. This synergy of diversity fuels a virtuous cycle of collaborative refinement, propelling algorithms towards greater robustness and adaptability in real-world scenarios. |
As the horizon of AI advancement expands, the unlocking of decentralized model training's potential heralds a renaissance in algorithmic evolution. Through decentralized orchestration and privacy-conscious collaboration, the power of collective intelligence becomes the cornerstone of AI innovation, paving the path towards a more equitable and resilient digital future.
Preserving Privacy in Collaborative AI
In the realm of cooperative artificial intelligence endeavors, safeguarding individual confidentiality emerges as a paramount concern. The essence of this endeavor lies in fortifying the sanctity of personal data, ensuring its inviolability throughout the collaborative process.
Upholding Anonymity: Within the sphere of collaborative AI initiatives, the assurance of anonymity serves as a cornerstone principle. By obfuscating individual identities and attributions, participants can engage in collective endeavors without compromising personal privacy.
Ensuring Data Confidentiality: The preservation of data confidentiality constitutes a fundamental tenet of collaborative AI endeavors. Through robust encryption mechanisms and stringent access controls, sensitive information remains shielded from unauthorized access or exploitation.
Embedding Privacy-Preserving Techniques: Integrating advanced cryptographic protocols and differential privacy mechanisms embeds an additional layer of protection within collaborative AI frameworks. These techniques enable the extraction of valuable insights while preserving the anonymity and confidentiality of individual contributions.
Cultivating Trust through Transparency: Fostering an environment of transparency fosters trust among participants in collaborative AI initiatives. By elucidating the methodologies employed to safeguard privacy, stakeholders can cultivate a sense of assurance regarding the integrity of the collaborative process.
Ensuring Data Security in Collaborative Intelligence Training with Privacy Preservation
To guarantee the integrity and confidentiality of sensitive information within the realm of collaborative intelligence enhancement without compromising individual privacy, robust measures must be implemented. This section delves into the multifaceted strategies aimed at fortifying the security of data in the collaborative refinement of intelligent systems while upholding the sanctity of personal privacy.
Encryption Protocols: Employing sophisticated encryption mechanisms stands as a cornerstone in safeguarding data integrity during the collaborative refinement of cognitive frameworks. By utilizing encryption protocols, sensitive information traversing network channels becomes indecipherable to unauthorized entities, thus mitigating the risk of illicit data access.
Secure Transmission Channels: Establishing secure channels for data transmission is imperative to preemptively thwart potential breaches during the collaborative enhancement of cognitive capabilities. Through the adoption of robust communication protocols and cryptographic techniques, the integrity of data exchanges is preserved, ensuring that sensitive information remains shielded from unauthorized interception.
Anonymization Techniques: Leveraging anonymization methodologies offers a proactive approach to preserving individual privacy amidst collaborative intelligence refinement endeavors. By anonymizing pertinent data points, the association between sensitive information and individual identities is obfuscated, thereby safeguarding personal privacy while facilitating effective collaboration in intelligence training.
Access Control Mechanisms: Implementing stringent access control mechanisms serves as a pivotal strategy in fortifying the security of data repositories utilized in collaborative cognitive enhancement initiatives. By delineating access privileges based on predefined criteria, unauthorized entities are precluded from infiltrating sensitive data repositories, thus mitigating the risk of unauthorized data access.
Audit Trails: Establishing comprehensive audit trails enables the meticulous tracking of data access and manipulation activities throughout the collaborative refinement of cognitive frameworks. By maintaining detailed records of user interactions with sensitive information, discrepancies and anomalous activities can be promptly identified and addressed, bolstering the overall security posture of collaborative intelligence training initiatives.
The Future of Confidential AI Advancement
In the forthcoming landscape of AI, the trajectory of safeguarding sensitive information while advancing computational intelligence is paramount. As technology strides towards greater sophistication, the imperative to fortify data privacy without compromising innovation becomes increasingly salient.
Rethinking Data Security Paradigms
Within the realm of artificial intelligence, the evolution of privacy-enhancing methodologies is poised to redefine conventional approaches to data security. In the forthcoming era, emphasis will be placed on fostering trust through robust encryption mechanisms, thereby ensuring the sanctity of individual information throughout the AI lifecycle.
The cornerstone of this paradigm shift lies in the integration of cutting-edge cryptographic protocols and decentralized architectures, which serve as bulwarks against unauthorized access and malicious exploitation. As the digital landscape expands, the imperative to fortify data sovereignty and preserve individual autonomy underscores the urgency of these advancements.