In recent years, there has been a significant advancement in the field of Artificial Intelligence (AI) and Augmented Reality (AR). These technologies have become increasingly popular and have the potential to enhance virtual experiences in various fields such as gaming, education, healthcare, and...
Revolutionizing Drug Discovery and Toxicity Testing with AI
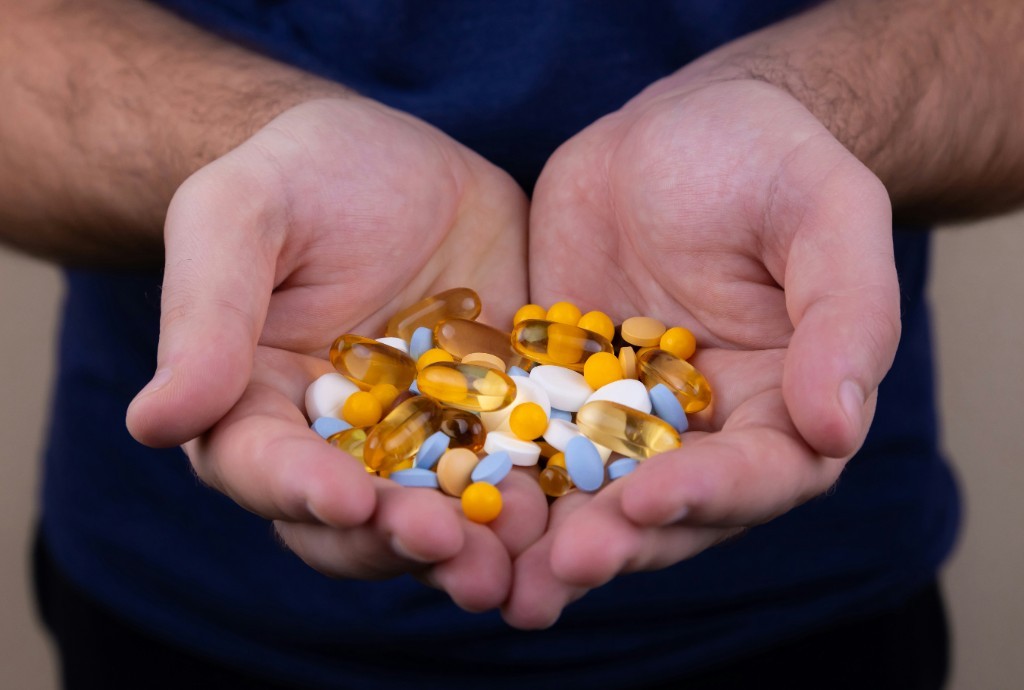
In recent years, the field of drug discovery and toxicity testing has seen a significant advancement with the integration of Artificial Intelligence (AI). AI has revolutionized the way drugs are developed and tested, making the process faster, more efficient, and more accurate.
Traditionally, drug discovery and toxicity testing have been time-consuming and expensive processes. Researchers would spend years screening thousands of compounds and conducting numerous experiments to identify potential drug candidates. However, with the help of AI, this process has been shortened considerably.
AI algorithms can analyze vast amounts of biological and chemical data, allowing researchers to identify potential drug candidates more quickly. These algorithms can predict the efficacy and safety of a drug, as well as its potential side effects. This not only saves time and resources but also reduces the need for animal testing.
Moreover, AI can also assist in the discovery of new drug targets. By analyzing large datasets and identifying patterns, AI algorithms can identify proteins or genes that may be potential targets for drug development. This opens up new possibilities for the treatment of various diseases.
Overall, the integration of AI into drug discovery and toxicity testing has the potential to revolutionize the pharmaceutical industry. With its ability to analyze large amounts of data and predict outcomes, AI can significantly speed up the drug development process, leading to the discovery of new and more effective treatments.
Overview of AI in Drug Discovery
Artificial intelligence (AI) is revolutionizing the field of drug discovery by accelerating the identification and development of new drugs. With the growing complexity of diseases and the need for more effective treatments, AI offers immense potential to transform the pharmaceutical industry.
Using AI for Target Identification
One of the key areas where AI is making a significant impact is in target identification. Traditional drug discovery methods involve a lengthy and expensive trial-and-error process. AI algorithms, on the other hand, can analyze large datasets and identify potential drug targets with higher accuracy and efficiency. By leveraging machine learning and data mining techniques, AI can identify patterns and relationships in biological data that humans may have missed.
Accelerating Drug Design and Optimization
Another area where AI is proving valuable is in drug design and optimization. AI algorithms can generate and screen millions of virtual compounds to identify those with the highest likelihood of success. This enables researchers to explore a much larger chemical space and discover novel drug candidates more quickly. AI can also optimize the properties of existing drugs, such as their efficacy and safety profiles, by predicting their interactions with target molecules.
In addition to target identification and drug design, AI is also being used in toxicity testing, clinical trial optimization, and personalized medicine. By leveraging AI technologies, researchers and pharmaceutical companies can streamline the drug discovery process, reduce costs, and ultimately bring safer and more effective drugs to market faster.
Benefits of Using AI in Drug Discovery
Artificial Intelligence (AI) has revolutionized the field of drug discovery, offering numerous benefits and opportunities for pharmaceutical research. Here are some key advantages of using AI in drug discovery:
- Accelerated Drug Discovery Process: AI algorithms can quickly analyze vast amounts of data and identify potential drug candidates with high accuracy. This speeds up the drug discovery process, which can otherwise take years using traditional methods.
- Improved Target Identification: AI algorithms can analyze complex biological datasets to identify potential drug targets and pathways, leading to the development of more effective and targeted therapies.
- Enhanced Lead Optimization: AI algorithms can predict the properties and behavior of drug molecules, helping researchers optimize lead compounds for improved efficacy and reduced toxicity.
- Reduced Cost and Time: AI can significantly reduce the cost and time required for drug discovery by automating various time-consuming tasks, such as data analysis and virtual screening.
- Increased Success Rate: By leveraging AI, researchers can make more informed decisions about which drug candidates to pursue, increasing the likelihood of success in clinical trials and ultimately bringing more effective drugs to market.
- Improved Safety and Toxicity Testing: AI algorithms can predict the potential toxicity of drug candidates, allowing researchers to prioritize safer compounds and reduce the risk of adverse effects in patients.
- Exploration of Novel Drug Targets: AI can help identify novel drug targets and pathways that may have been overlooked using traditional methods, opening up new avenues for drug discovery and innovation.
- Personalized Medicine: By analyzing large-scale patient data, AI can help identify patient subgroups that may benefit from specific treatments, enabling the development of personalized medicine approaches.
In conclusion, AI has the potential to revolutionize the field of drug discovery by accelerating the process, improving target identification, optimizing lead compounds, reducing costs and time, increasing success rates, improving safety and toxicity testing, exploring novel drug targets, and enabling personalized medicine. With continued advancements in AI technology, the future of drug discovery looks promising and holds great potential for the development of more effective and targeted therapies.
Challenges and Limitations of AI in Drug Discovery
Artificial Intelligence (AI) has emerged as a powerful tool in the field of drug discovery, offering the potential to accelerate the process of identifying novel drug candidates and predicting their toxicity. However, there are several challenges and limitations that need to be addressed in order to fully leverage the potential of AI in this domain.
One of the major challenges is the availability of high-quality data. AI algorithms rely on large datasets to learn patterns and make accurate predictions. However, in the field of drug discovery, data is often scarce and fragmented. This makes it difficult for AI models to learn effectively and limits their predictive power. Therefore, efforts should be made to improve data sharing and collaboration among researchers to overcome this limitation.
Another challenge is the interpretability of AI models. While AI algorithms can generate accurate predictions, they often lack transparency in their decision-making process. This makes it difficult for researchers to understand how the models arrive at their conclusions, hindering the validation and acceptance of AI-generated results in the drug discovery pipeline. Developing interpretable AI models is crucial to gain trust and confidence in their predictions.
Furthermore, the complexity of biological systems poses a significant challenge for AI in drug discovery. Biological processes are intricate and multifaceted, involving numerous interactions and pathways. AI models need to capture this complexity in order to accurately predict drug-target interactions and toxicity. Developing more sophisticated AI algorithms that can handle this complexity is an ongoing area of research.
Additionally, the lack of domain expertise in AI is another limitation. Drug discovery requires deep knowledge of biology, chemistry, and pharmacology. AI experts often lack this domain expertise, which can hinder the successful application of AI in drug discovery. Collaborations between AI experts and domain experts are essential to ensure that AI tools are designed and utilized effectively in this field.
Lastly, there are regulatory and ethical challenges associated with the use of AI in drug discovery. Regulatory agencies have yet to establish clear guidelines for the validation and approval of AI-generated results. Ethical concerns regarding data privacy, bias, and accountability also need to be addressed. Developing regulatory frameworks and ethical guidelines specific to AI in drug discovery is crucial to ensure responsible and safe use of AI technologies.
AI in Toxicity Testing
Artificial Intelligence (AI) has revolutionized the field of toxicity testing by providing faster and more accurate predictions of chemical safety. Toxicity testing is crucial in drug discovery and development, as well as in assessing the safety of chemicals used in various industries.
Improved Prediction Accuracy
One of the main advantages of AI in toxicity testing is its ability to improve prediction accuracy. Traditional toxicity testing methods rely on animal models, which are time-consuming, expensive, and may not accurately represent human responses. AI models can analyze large datasets of chemical structures, biological data, and toxicity outcomes to identify patterns and predict toxicity levels with higher accuracy.
AI models can also consider various factors that influence toxicity, such as chemical structure, molecular properties, and biological pathways. This multidimensional approach allows for a more comprehensive assessment of potential toxicity, leading to better decision-making in drug discovery and chemical safety evaluation.
High-Speed Screening
Another benefit of AI in toxicity testing is its ability to accelerate the screening process. Traditional toxicity testing methods require extensive experimentation and evaluation, which can be time-consuming and costly. AI models can quickly analyze vast amounts of data and provide toxicity predictions in a fraction of the time.
This speed allows researchers to prioritize the most promising compounds for further testing, saving time and resources. Additionally, AI models can identify potential toxic compounds early in the drug discovery process, reducing the likelihood of investing in unsuccessful drug candidates.
Moreover, AI can facilitate high-throughput screening, where thousands of compounds can be tested simultaneously. This capability is especially valuable in the context of large chemical libraries, where AI can efficiently identify compounds with potential toxicity concerns.
In conclusion, AI has greatly enhanced toxicity testing by improving prediction accuracy and speeding up the screening process. These advancements have the potential to accelerate drug discovery, reduce animal testing, and ensure the safety of chemicals used in various applications.
Advantages of AI in Toxicity Testing
Toxicity testing is a critical step in drug discovery and development, as it helps to identify and evaluate the potential adverse effects of new compounds. Traditionally, toxicity testing has been performed using animal models, which can be time-consuming, expensive, and ethically controversial. However, the emergence of artificial intelligence (AI) has revolutionized the field of toxicity testing, offering several advantages over traditional methods.
1. Faster and more efficient screening
AI algorithms can analyze large datasets and identify patterns and trends much faster than human researchers. This enables the screening of thousands, or even millions, of compounds in a fraction of the time it would take using traditional methods. By accelerating the screening process, AI can help to identify potential toxic compounds earlier in the drug discovery pipeline, saving time and resources.
2. Reduced reliance on animal testing
One of the major ethical concerns in drug development is the use of animal models for toxicity testing. AI has the potential to significantly reduce the reliance on animal testing by providing alternative methods for toxicity assessment. By utilizing in silico modeling, AI algorithms can predict the toxicity of compounds based on their chemical structure and properties, eliminating the need for animal experiments in certain cases.
Moreover, AI can incorporate data from previous toxicity studies to refine and improve its predictions, leading to more accurate and reliable results. This not only reduces the number of animals used for testing but also provides a more comprehensive understanding of toxicity mechanisms.
Overall, AI has the potential to revolutionize toxicity testing in drug discovery by offering faster, more efficient screening methods and reducing the reliance on animal testing. By harnessing the power of AI, researchers can accelerate the identification of safe and effective drug candidates, ultimately benefiting both patients and the environment.