In recent years, there has been a significant advancement in the field of Artificial Intelligence (AI) and Augmented Reality (AR). These technologies have become increasingly popular and have the potential to enhance virtual experiences in various fields such as gaming, education, healthcare, and...
Robot Learning Through Motion and Object Interaction - Unlocking the Potential of AI Technology
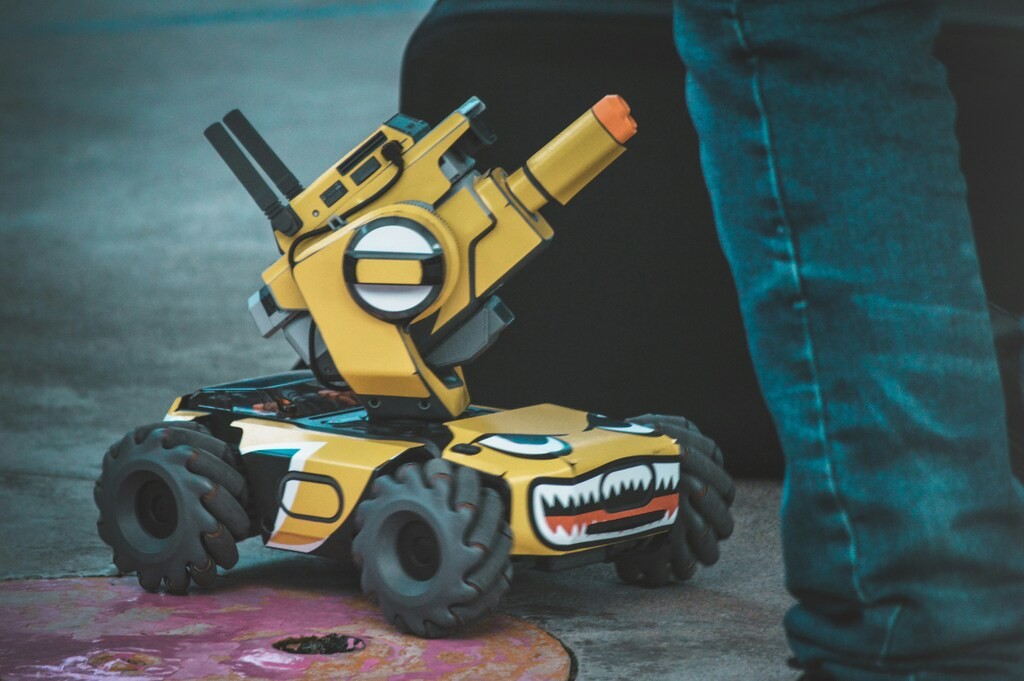
In recent years, there have been significant advancements in the field of robot learning through motion and object interaction. Robots are no longer limited to performing repetitive tasks; they are now capable of adapting and learning from their environment. This has opened up new possibilities for robots to interact with objects and humans in more intelligent and intuitive ways.
One key area of research in robot learning is motion planning. Robots are now able to generate their own motion plans to navigate through complex environments. This allows them to avoid obstacles and reach their target locations more efficiently. By learning from their past experiences, robots can also improve their motion planning abilities over time, becoming more adept at handling new situations and challenges.
Another important aspect of robot learning is object interaction. Robots can now learn to manipulate objects with their robotic arms, using techniques such as grasping, lifting, and placing. This opens up a wide range of applications, from assembly line tasks to household chores. By learning from their interactions with objects, robots can improve their manipulation skills and become more proficient at performing complex tasks.
Advancements in robot learning through motion and object interaction have the potential to revolutionize various industries. In manufacturing, robots can now take on more complex tasks, increasing productivity and efficiency. In healthcare, robots can assist with patient care and perform delicate surgical procedures with precision. In everyday life, robots can help with household chores and provide companionship for the elderly. The possibilities are endless.
As researchers continue to push the boundaries of robot learning, we can expect even more impressive advancements in the future. Robots will become increasingly capable of learning from their interactions with the world, adapting to new situations, and collaborating with humans. This will enable them to become valuable partners in various industries and enhance our daily lives in ways we never thought possible.
Advancements in Robot Learning
Robot learning has made significant advancements in recent years, particularly in the areas of motion and object interaction. These advancements have paved the way for robots to become more autonomous and capable of performing complex tasks.
One major area of advancement is in the development of algorithms and techniques that allow robots to learn and adapt through interaction with their environment. By observing and interacting with objects, robots can learn how to manipulate them and perform tasks with greater efficiency and accuracy.
Another significant advancement is the use of deep learning and neural networks in robot learning. These techniques allow robots to process and analyze large amounts of data, enabling them to recognize patterns and make predictions based on previous experiences.
Advancements in robot learning have also led to improvements in robot manipulation and grasping. Through the use of advanced sensors and algorithms, robots can now accurately and securely grasp objects of various shapes and sizes, even in complex and dynamic environments.
Furthermore, robot learning has also advanced in the field of motion planning. Robots can now generate efficient and smooth trajectories to navigate through cluttered environments, avoiding obstacles and reaching their goals with minimal effort.
Advancements in Robot Learning:
- Learning through interaction with the environment
- Deep learning and neural networks
- Improved robot manipulation and grasping
- Advanced motion planning
Overall, the advancements in robot learning have opened up new possibilities for robots to operate in a wide range of industries and applications. With continued research and development, robots will become even more capable and autonomous, revolutionizing the way we interact with technology.
Motion-Based Learning
Motion-based learning is a significant area of research in the field of robot learning. It focuses on enabling robots to learn and adapt their behavior through interaction with their environment and objects.
This approach allows robots to acquire new skills and improve their performance by observing and imitating human actions or by exploring their surroundings. By analyzing the motion data and object interactions, robots can learn to perform complex tasks and manipulate objects with precision.
One of the main challenges in motion-based learning is developing algorithms and models that can accurately interpret and understand human actions. This involves extracting meaningful information from the raw motion data, such as joint angles, velocities, and trajectories.
Another important aspect of motion-based learning is the ability to generalize learned behaviors to new scenarios and objects. This requires robots to understand the underlying principles and constraints of the task and to adapt their actions accordingly.
In recent years, advancements in machine learning and computer vision have greatly contributed to the progress of motion-based learning. Deep learning algorithms, such as convolutional neural networks (CNNs) and recurrent neural networks (RNNs), have been successfully applied to analyze motion data and recognize human actions.
Motion-based learning has numerous applications in various fields, including robotics, healthcare, entertainment, and manufacturing. For example, robots equipped with motion-based learning capabilities can assist humans in performing complex tasks, such as assembling or operating machinery.
In conclusion, motion-based learning plays a crucial role in advancing the capabilities of robots. It allows them to learn from their interactions with the environment and objects, enabling them to perform complex tasks and adapt to new scenarios. With further advancements in machine learning and computer vision, motion-based learning will continue to drive innovation in the field of robotics.
Object Interaction and Learning
One of the key advancements in robot learning is the ability to interact with objects. By understanding how to manipulate and interact with objects, robots can perform a wide range of tasks in various environments. This has been made possible through advancements in machine learning algorithms and sensor technologies.
Learning Object Affordances
One important aspect of object interaction is learning object affordances. Affordances refer to the potential actions or uses of an object based on its physical properties. For example, a cup can be used to hold liquids or can be grasped by a robot. By learning these affordances, robots can understand how to interact with objects and perform tasks based on their intended use.
Machine learning algorithms have been developed to enable robots to learn object affordances. These algorithms analyze the physical properties of objects, such as shape, size, and material, and learn the potential actions that can be performed with them. By training on a large dataset of object interactions, robots can generalize their knowledge and apply it to new objects they encounter.
Learning Object Manipulation
In addition to learning object affordances, robots can also learn how to manipulate objects. This involves understanding the physics of object manipulation, such as grasping, lifting, and moving objects. By learning these skills, robots can perform complex tasks such as picking up objects, sorting them, and assembling them.
Learning object manipulation involves training robots on a combination of simulation and real-world data. Simulations allow robots to practice manipulating objects in a controlled environment, while real-world data provides the necessary variability and complexity. By combining these two sources of data, robots can learn how to manipulate objects in a wide range of scenarios.
In conclusion, object interaction and learning are crucial advancements in robot learning. By understanding object affordances and learning object manipulation, robots can perform a wide range of tasks and adapt to different environments. These advancements have been made possible through the development of machine learning algorithms and sensor technologies, and will continue to drive the progress of robot learning in the future.
Emerging Trends in Robot Learning
In recent years, there have been significant advancements in the field of robot learning. As robots become more sophisticated and capable of interacting with their environment, new trends are emerging that promise to revolutionize the way robots learn and adapt.
1. Reinforcement Learning
One of the most promising trends in robot learning is the use of reinforcement learning. This approach involves training robots through a system of rewards and punishments, similar to how humans learn from trial and error. By using reinforcement learning algorithms, robots can learn complex tasks and behaviors through interaction with their environment.
Reinforcement learning has the potential to greatly improve the autonomy and adaptability of robots. By allowing robots to learn from their own experiences, they can develop strategies and decision-making skills that were previously only possible through explicit programming.
2. Interactive Learning
Another emerging trend in robot learning is interactive learning. This approach involves robots actively seeking out and engaging with humans or other objects in their environment to learn new tasks or behaviors.
Interactive learning allows robots to learn from human demonstrations or through collaborative interactions. By observing and imitating human actions, robots can quickly acquire new skills and knowledge. This trend is particularly beneficial in domains where explicit programming or pre-training is difficult or time-consuming.
Furthermore, interactive learning promotes human-robot collaboration and can lead to more intuitive and natural interactions between humans and robots. This has significant implications for the development of robots that can seamlessly integrate into our daily lives.
In conclusion, the field of robot learning is constantly evolving, and new trends are emerging that promise to enhance the capabilities of robots. Reinforcement learning and interactive learning are two particularly promising trends that have the potential to revolutionize the way robots learn and adapt. As these trends continue to develop, we can expect to see even more sophisticated and capable robots in the future.