In recent years, there has been a significant advancement in the field of Artificial Intelligence (AI) and Augmented Reality (AR). These technologies have become increasingly popular and have the potential to enhance virtual experiences in various fields such as gaming, education, healthcare, and...
Streamlining AI Model Development with Automated Machine Learning (AutoML)
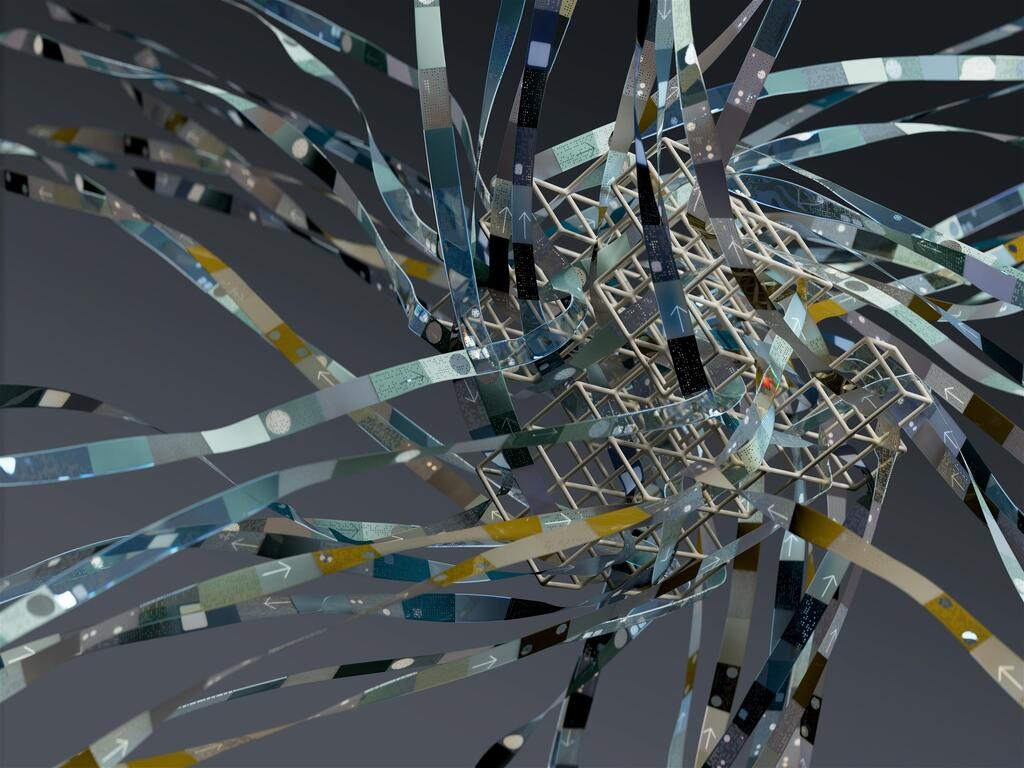
Innovative techniques are reshaping the landscape of AI model refinement, transcending traditional methodologies. This evolutionary process navigates the intricacies of algorithmic empowerment to elevate AI systems to unprecedented heights of efficiency and accuracy.
Within this realm of advancement, a paradigm shift is underway, ushering in a new era of automated optimization. Gone are the days of labor-intensive manual adjustments; instead, a harmonious fusion of computational prowess and adaptive intelligence propels AI models towards their zenith.
Unveiling the veil of complexity, this transformative journey empowers developers to unlock the full potential of AI systems, effortlessly fine-tuning performance metrics with precision and agility. Through an intricate dance of data orchestration and algorithmic finesse, the boundaries of what's achievable in AI are continually expanded.
Enhancing AI Model Evolution through Automated Learning Processes
In this segment, we delve into the realm of revolutionizing the augmentation of artificial intelligence frameworks via automated cognitive procedures. Through the integration of advanced algorithms and data-driven methodologies, we explore avenues to expedite the refinement and enhancement of AI models without manual intervention.
- Optimizing Cognitive Evolution: Unveiling the mechanisms behind accelerating the maturation of AI constructs through automated iterations.
- Facilitating Iterative Enhancements: Delving into strategies to foster continual improvement within artificial intelligence frameworks via autonomous learning cycles.
- Maximizing Efficiency in Augmentation: Exploring methodologies to maximize the efficiency and efficacy of AI model evolution through automated streams.
This section offers insights into leveraging cutting-edge technologies to propel the evolution of artificial intelligence paradigms, ushering in a new era of streamlined advancement.
Enhancing Efficiency Through Automated Model Selection
In the pursuit of optimizing the process of identifying the most suitable algorithms for a given task, the focus lies on enhancing efficiency through the automated selection of models. This approach aims to expedite the decision-making process by leveraging computational algorithms to discern the most appropriate methodologies for achieving desired outcomes.
Advantages of Automated Model Selection |
Challenges in Implementation |
1. Accelerates the model selection process |
1. Ensuring the compatibility of automated selection with diverse datasets |
2. Improves accuracy by minimizing human bias |
2. Addressing potential biases inherent in algorithmic decision-making |
3. Facilitates scalability by handling large datasets efficiently |
3. Overcoming complexity in integrating automated selection into existing workflows |
By harnessing the power of automated techniques, organizations can streamline the process of model selection, thereby allocating resources more effectively and ultimately enhancing the overall efficiency of AI-driven endeavors.
Optimizing Hyperparameters Automatically for Enhanced Performance
In the pursuit of refining the efficacy of AI algorithms, a pivotal aspect lies in the meticulous calibration of hyperparameters. These parameters, akin to the dials and knobs of a complex machinery, wield significant influence over the performance and behavior of AI models. However, the manual tuning of these hyperparameters is a labor-intensive endeavor fraught with challenges. To circumvent these hurdles and elevate the efficiency of AI systems, the process of automatically optimizing hyperparameters emerges as a beacon of innovation.
Automating the optimization of hyperparameters entails the orchestration of algorithms that dynamically adjust these configuration variables, steering the model towards optimal performance thresholds. This method liberates practitioners from the arduous task of hand-tuning parameters, allowing them to allocate their cognitive resources towards more creative and strategic pursuits.
By harnessing the power of computational algorithms, this approach navigates through the labyrinthine landscape of hyperparameter space, traversing the myriad configurations to pinpoint the settings that yield maximal performance gains. Through iterative exploration and refinement, automated hyperparameter optimization unleashes the latent potential of AI models, enhancing their predictive accuracy and generalization capabilities.
- Efficiently navigating hyperparameter space
- Liberating practitioners from manual tuning
- Maximizing predictive accuracy and generalization
Simplifying Data Preprocessing with Advanced Automation Pipelines
Data preprocessing stands as the foundation for robust and accurate AI model construction. Its significance lies in cleansing, transforming, and organizing raw data to a form suitable for analysis and modeling. In the realm of modern AI advancement, the utilization of advanced automation pipelines revolutionizes this crucial stage, enabling a seamless and efficient process from raw data to insightful model input.
Enhanced Efficiency: Traditional data preprocessing methods often entail manual labor and intricate programming, consuming significant time and resources. However, with the integration of advanced automation pipelines, the cumbersome tasks of data cleaning, feature engineering, and normalization are streamlined, reducing human intervention and accelerating the overall model development process.
Intelligent Data Handling: AutoML pipelines incorporate intelligent algorithms and techniques to handle diverse data types and formats effectively. From handling missing values to encoding categorical variables, these pipelines adapt dynamically to the unique characteristics of the dataset, ensuring comprehensive data preprocessing tailored to the specific requirements of the AI model.
Optimized Workflow: By automating data preprocessing tasks, AutoML pipelines not only enhance efficiency but also optimize the overall workflow of AI model development. The seamless integration of data preprocessing with subsequent stages such as feature selection and model training fosters a cohesive and iterative approach, facilitating rapid experimentation and refinement of AI models.
Empowering Data Scientists: With the complexities of data preprocessing abstracted through automation, data scientists can redirect their focus towards higher-level tasks such as model interpretation, validation, and deployment. By alleviating the burden of manual data manipulation, AutoML pipelines empower data scientists to delve deeper into the intricacies of AI model design and application.
Conclusion: In the landscape of AI innovation, the integration of advanced automation pipelines heralds a new era of simplification and efficiency in data preprocessing. By harnessing intelligent algorithms and techniques, these pipelines offer a transformative solution to the challenges of data preparation, paving the way for accelerated AI model development and deployment.