In recent years, there has been a significant advancement in the field of Artificial Intelligence (AI) and Augmented Reality (AR). These technologies have become increasingly popular and have the potential to enhance virtual experiences in various fields such as gaming, education, healthcare, and...
Streamlining Deep Learning Model Design with Neural Architecture Search Automation
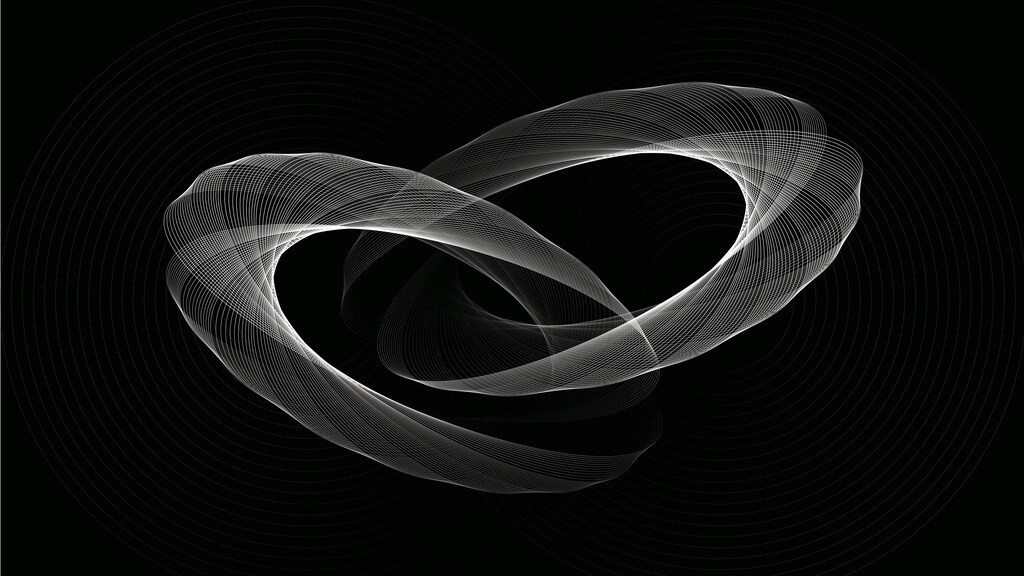
In the ever-evolving realm of cognitive frameworks, a burgeoning approach has emerged to streamline the intricate process of crafting sophisticated mental structures. This innovative methodology transcends conventional paradigms, harnessing the prowess of computational intelligence to sculpt intricate networks of cerebral connectivity.
Exploring the frontier of cognitive synthesis, this paradigmatic shift leverages automated methodologies to orchestrate the emergence of intricate neural configurations. Through a symphony of algorithmic ingenuity, this transformative approach imbues machines with the capacity for autonomous evolution, birthing a new era of cognitive prowess.
Unshackled from the constraints of manual intervention, this avant-garde technique empowers systems to iteratively refine and augment their cognitive blueprints. By navigating the labyrinthine landscape of potential configurations, these algorithms traverse the vast expanse of possibility, sculpting architectures that resonate with unprecedented efficiency and efficacy.
Revolutionizing Innovative Creation of Complex Cognitive Systems
In the ever-evolving landscape of intelligent technology, a paradigm shift is underway in how we conceive, construct, and refine sophisticated cognitive frameworks. This transformative movement transcends conventional methodologies, ushering in an era where the genesis of intricate neural structures is redefined.
Redefining Creativity in Computational Systems
Unlocking the potential of computational intelligence demands a departure from traditional frameworks. By revolutionizing the genesis of intricate neural structures, we embark on a journey towards unprecedented creativity in the realm of computational systems. Gone are the days of manual intervention; instead, algorithms take the helm, navigating the labyrinth of possibilities with unparalleled efficiency.
- Embracing Algorithmic Innovation: Delving into the realm of algorithmic innovation, we witness a departure from static architectures towards dynamic, evolving models. Through iterative exploration, algorithms uncover novel pathways, fostering the emergence of cutting-edge cognitive systems.
- Empowering Evolutionary Progression: Harnessing the power of evolutionary algorithms, the process of model creation becomes a journey of self-improvement. As models adapt, mutate, and evolve, they transcend limitations, paving the way for a new era of cognitive prowess.
- Fostering Collaborative Intelligence: By fostering synergy between algorithms and human intuition, a new paradigm of collaborative intelligence emerges. This symbiotic relationship unlocks the collective potential of human ingenuity and computational prowess, propelling us towards uncharted frontiers of innovation.
Embracing the Evolution of Cognitive Constructs
The evolution of cognitive constructs heralds a new dawn in intelligent technology. Through the amalgamation of algorithmic prowess and human ingenuity, we transcend the boundaries of conventional thinking, paving the way for transformative advancements in the field of computational intelligence.
Exploring the Landscape of Discovering Computational Structures
In this segment, we embark on an expedition into the diverse realm of uncovering computational blueprints. Our journey delves into the intricate terrain where algorithms traverse to uncover optimal arrangements, navigating through the vast expanse of potential configurations.
Navigating the Terrain
As we venture forth, we encounter a rich tapestry of methodologies aimed at unraveling the enigmatic patterns within data. Through systematic exploration and analysis, researchers seek pathways to efficiently navigate the landscape, forging novel routes toward superior computational frameworks.
Exploring Fertile Grounds
Our exploration extends beyond mere observation, as we actively engage with the fertile grounds of experimentation. Through rigorous evaluation and experimentation, we unearth insights that illuminate promising avenues for the advancement of computational intelligence.
Efficiency and Scalability in Model Configuration Streamlining
In the pursuit of refining the process of constructing and optimizing neural networks, a paramount focus lies on enhancing efficiency and scalability. This section delves into the strategies and methodologies employed to streamline the configuration of models, ensuring swift and adaptable frameworks.
- Enhancing Computational Efficiency
- Efforts are directed towards optimizing computational resources, reducing redundancy, and maximizing the utility of available hardware. Techniques such as parameter sharing, weight pruning, and quantization play pivotal roles in curtailing computational overhead.Scalability Through Modularization:
- Modular design principles facilitate scalability by compartmentalizing components of the model, enabling easy integration of new functionalities and adaptations to varying tasks and datasets. By abstracting intricate functionalities into modular units, scalability becomes an inherent characteristic of the model design process.Adaptive Resource Allocation:
- The dynamic allocation of resources, including memory, computation, and storage, is essential for accommodating diverse model architectures and datasets. Techniques such as dynamic batching, memory pooling, and adaptive learning rates aid in optimizing resource allocation based on the specific requirements of the model and the underlying hardware infrastructure.Efficient Hyperparameter Optimization:
Hyperparameters play a crucial role in determining the performance and generalization capability of neural networks. Efficient techniques for hyperparameter optimization, such as Bayesian optimization, genetic algorithms, and reinforcement learning, are instrumental in automating the process of hyperparameter tuning, thereby enhancing the efficiency of model configuration.