In recent years, there has been a significant advancement in the field of Artificial Intelligence (AI) and Augmented Reality (AR). These technologies have become increasingly popular and have the potential to enhance virtual experiences in various fields such as gaming, education, healthcare, and...
The Evolution of Artificial Intelligence: How Machines Are Enhancing Their Learning Abilities
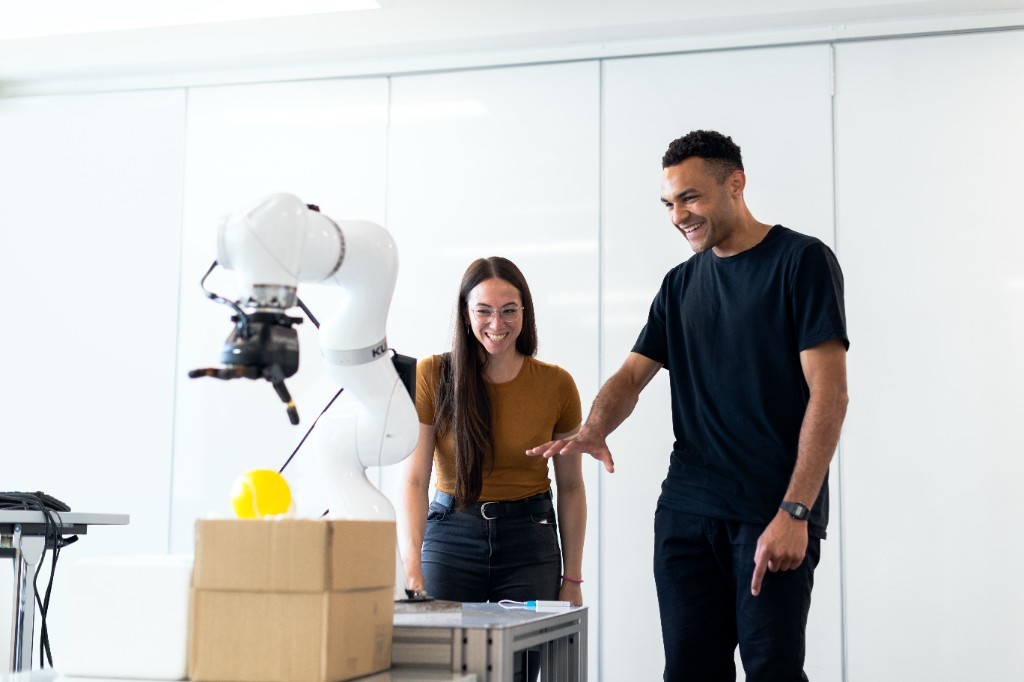
Artificial Intelligence (AI) has become an integral part of our daily lives, from voice assistants like Siri and Alexa to recommendation systems on platforms like Netflix and Amazon. But how do these machines learn and become smarter over time?
AI systems are designed to learn from data and improve their performance through experience. This process is known as machine learning. Instead of being explicitly programmed to perform certain tasks, machines are trained to recognize patterns and make predictions based on the data they are exposed to.
Machine learning algorithms play a key role in this process. These algorithms are designed to analyze large amounts of data and identify patterns or relationships that humans may not be able to detect. By processing massive datasets, machines can learn to make accurate predictions and decisions.
One common technique used in machine learning is supervised learning. In supervised learning, an AI system is trained on a labeled dataset, where each data point is associated with a specific outcome. The machine learns to recognize patterns in the data and make predictions based on these patterns.
For example, in a supervised learning model for image recognition, the machine is trained on a dataset of images, where each image is labeled with the object it contains. By analyzing the patterns in the labeled images, the machine can learn to accurately identify objects in new, unlabeled images.
Unsupervised learning is another approach used in machine learning. In unsupervised learning, the AI system is not provided with labeled data. Instead, it is tasked with finding patterns or relationships in the data on its own.
This approach is particularly useful when the AI system needs to discover hidden structures or clusters in the data that may not be obvious to humans.
For example, in unsupervised learning, an AI system could be used to analyze customer data and identify distinct groups of customers based on their purchasing behavior. This information can then be used to create targeted marketing campaigns for each customer segment.
By combining these and other machine learning techniques, AI systems are able to learn and adapt to new information, making them increasingly intelligent and capable of performing complex tasks. As machines continue to learn and improve, the potential applications of AI in various industries are expanding, from healthcare and finance to transportation and entertainment.
The Evolution of Artificial Intelligence
Artificial Intelligence (AI) has come a long way since its inception. Over the years, AI has evolved from simple rule-based systems to more complex machine learning algorithms that can learn from data and improve their performance over time. This evolution has been driven by advancements in computing power, availability of big data, and breakthroughs in algorithms.
In the early days of AI, researchers focused on developing expert systems, which were rule-based systems designed to mimic human intelligence in specific domains. These systems were limited in their ability to learn and adapt to new situations, as they relied on predefined rules and lacked the ability to generalize from data.
However, with the advent of machine learning, AI systems became more capable of learning from data. Machine learning algorithms, such as neural networks, were developed to enable computers to learn from examples and make predictions or decisions based on the patterns they discovered in the data. This marked a significant shift in the field of AI, as machines were no longer limited to following predefined rules but could now learn and improve their performance through experience.
As machine learning algorithms became more sophisticated, researchers began exploring deep learning, a subset of machine learning that involves training artificial neural networks with multiple layers. Deep learning has revolutionized many areas of AI, such as computer vision and natural language processing, enabling machines to recognize and interpret complex patterns in images and text.
Another important development in the evolution of AI is the rise of reinforcement learning. Reinforcement learning involves training an AI agent through trial and error, rewarding it for good actions and punishing it for bad actions. This approach has been used to develop AI systems that can play complex games, such as Go and chess, at a level surpassing human experts.
Looking ahead, the future of AI promises even greater advancements. Researchers are exploring new techniques, such as transfer learning, which allows AI systems to apply knowledge learned in one domain to another domain. This could greatly enhance the ability of AI systems to learn and adapt to new tasks and environments.
In conclusion, the evolution of AI has been driven by advancements in computing power, availability of big data, and breakthroughs in algorithms. From simple rule-based systems to complex machine learning algorithms, AI has become increasingly capable of learning and adapting to new situations. With ongoing research and development, AI is poised to continue its evolution and revolutionize various industries and sectors.