In recent years, there has been a significant advancement in the field of Artificial Intelligence (AI) and Augmented Reality (AR). These technologies have become increasingly popular and have the potential to enhance virtual experiences in various fields such as gaming, education, healthcare, and...
Unlocking AI Potential - Streamlining Hyperparameter Tuning with Scalable Bayesian Optimization
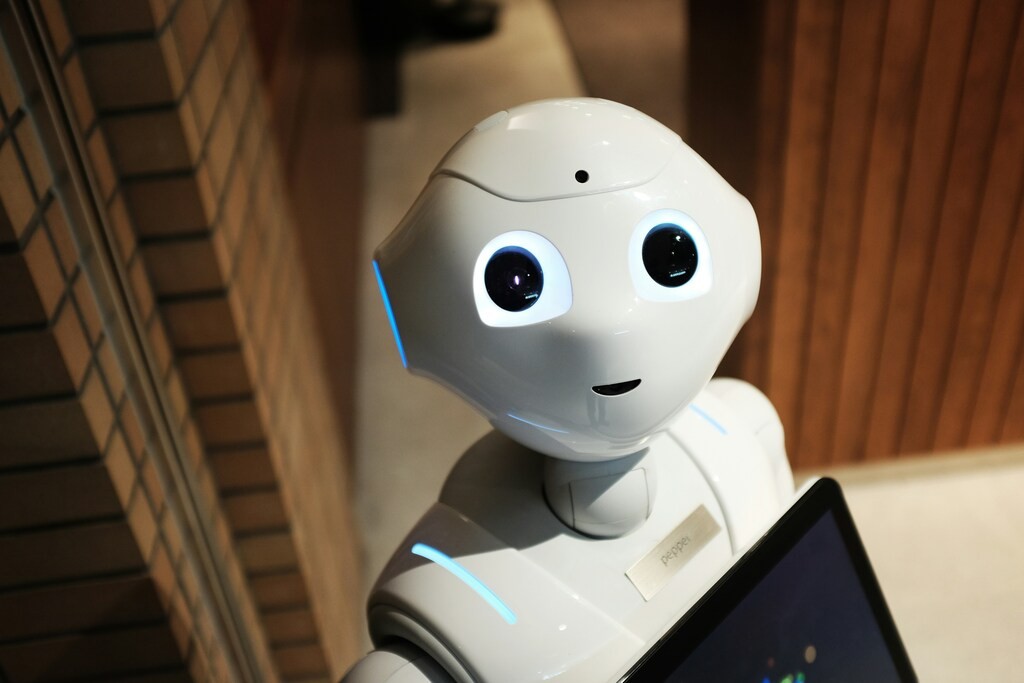
Unlocking the full potential of artificial intelligence hinges upon refining its intricate parameters to achieve optimal performance. In the realm of AI, the quest for efficiency in parameter adjustment is perpetual, driven by the pursuit of superior models and algorithms. This article delves into a sophisticated methodology poised to revolutionize the fine-tuning process, facilitating swift and precise adjustments to model configurations.
Redefining the Approach: Traditional methods for parameter refinement often encounter scalability challenges, impeding the seamless optimization of AI systems. This innovative framework introduces a paradigm shift in the optimization landscape, prioritizing adaptability and scalability without compromising efficacy. By leveraging advanced techniques rooted in probabilistic inference, this approach aims to revolutionize the trajectory of AI parameter optimization.
A New Frontier in AI Optimization: Harnessing the power of probabilistic reasoning, this methodology seeks to transcend the limitations of conventional optimization strategies. Through the seamless integration of statistical modeling and iterative refinement, it endeavors to navigate the vast parameter space with unparalleled agility and precision. The synergy between computational efficiency and Bayesian principles heralds a new era in AI optimization, promising enhanced performance across diverse applications.
Enhancing AI Performance with Scalable Bayesian Optimization
In the realm of artificial intelligence advancement, maximizing efficacy stands as a paramount pursuit. This section delves into strategies aimed at refining AI performance through a systematic approach that harnesses the power of adaptable methodologies. By leveraging scalable techniques rooted in probabilistic inference and iterative refinement, this discourse unveils a transformative pathway towards elevating the operational efficiency of AI systems.
Unlocking Potential through Iterative Refinement: Central to enhancing AI performance lies the iterative process of refinement, wherein the intrinsic capabilities of the system are progressively honed. Through a nuanced exploration of diverse strategies, the endeavor unfolds as a journey towards uncovering latent potential and optimizing resource allocation.
Harnessing Probabilistic Inference: The integration of probabilistic inference furnishes a foundational framework for navigating the complexities inherent in AI optimization. By embracing the inherent uncertainty within datasets and computational models, this approach facilitates informed decision-making, paving the way for robust performance enhancements.
Adaptable Methodologies for Dynamic Environments: In the dynamic landscape of AI deployment, adaptability emerges as a cornerstone principle. The adoption of scalable methodologies ensures resilience in the face of evolving challenges, enabling seamless adjustments to varying contexts and operational demands.
Driving Innovation through Systematic Exploration: At the heart of performance enhancement lies a commitment to systematic exploration and experimentation. By cultivating an environment conducive to innovation, AI systems stand poised to transcend existing limitations and propel towards unprecedented levels of efficacy.
Conclusion: In the pursuit of augmenting AI performance, the integration of scalable Bayesian optimization methodologies offers a transformative paradigm. Through iterative refinement, probabilistic inference, and adaptability, the trajectory towards optimal performance is illuminated, promising a future where AI systems operate at the pinnacle of efficiency.
Streamlining Hyperparameter Adjustment for Deep Learning Models
Enhancing the performance of deep learning models hinges significantly on meticulous calibration of various parameters. In this section, we delve into strategies aimed at refining the process of parameter adjustment, facilitating smoother optimization of model performance.
Understanding the Complexity of Hyperparameter Configuration
The journey towards optimal model performance is often obstructed by the intricate nature of hyperparameter configuration. Deep learning models, with their multitude of parameters, pose a formidable challenge in finding the right balance between performance and efficiency.
- Exploring Automated Techniques: Leveraging automated methodologies to navigate the vast space of hyperparameters efficiently.
- Embracing Intuitive Heuristics: Incorporating intuitive rules and guidelines to streamline the process of hyperparameter adjustment.
- Iterative Refinement: Adopting iterative approaches to progressively fine-tune hyperparameters based on evolving insights gained from model performance.
By employing a combination of automated techniques, intuitive heuristics, and iterative refinement, the arduous task of hyperparameter adjustment can be simplified, paving the way for more effective deep learning model optimization.
Revolutionizing AI Model Optimization with Scalable Bayesian Methods
In the realm of enhancing AI model performance, a paradigm shift is underway, propelled by innovative approaches that transcend traditional optimization methodologies. This section delves into the transformative landscape of AI model optimization, driven by the integration of advanced, adaptable Bayesian strategies. Rather than relying on conventional methods, this evolutionary leap harnesses the power of scalable Bayesian techniques to navigate the intricate terrain of model refinement.
At its core, this revolution is characterized by a departure from rigid optimization frameworks towards dynamic, responsive methodologies. By embracing scalable Bayesian methods, practitioners unlock a realm of possibilities where model enhancement becomes a fluid, iterative process. Gone are the days of static tuning parameters; instead, a nuanced exploration of model space ensues, guided by probabilistic reasoning and adaptive learning.
- Embracing Dynamic Model Enhancement
- Probabilistic Exploration of Model Space
- Fostering Iterative Refinement
- Adaptive Learning Paradigms
- Navigating Complexity with Bayesian Ingenuity
This section illuminates the profound impact of scalable Bayesian methods on the optimization landscape, heralding a new era where AI models evolve symbiotically with their environments. Through dynamic exploration, probabilistic reasoning, and adaptive learning, the journey towards optimal model performance transcends mere efficiency, embracing a holistic approach to intelligence augmentation.
Efficiently Tailoring Hyperparameters for Enhanced Performance
To effectively optimize the parameters influencing performance in AI systems, a meticulous approach is indispensable. This section delves into strategies aimed at fine-tuning the pivotal elements that govern the efficacy of AI models. By adeptly adjusting these factors, the performance can be significantly bolstered, leading to superior outcomes.
Customizing Parameters for Optimal Results
In the pursuit of attaining optimal performance, it becomes imperative to tailor the parameters judiciously. This entails a nuanced examination of the various elements that contribute to the model's functionality, allowing for meticulous adjustments to be made. By customizing these parameters with precision, the model can exhibit heightened efficiency and efficacy.
Refining Model Configuration for Enhanced Functionality
Refinement of model configuration stands as a cornerstone in the quest for superior performance. Through meticulous calibration of the parameters, the model's capacity to discern patterns and make informed decisions can be substantially enhanced. This iterative process of refinement enables the model to adapt dynamically to diverse datasets and tasks, thereby augmenting its overall functionality.
Strategic Parameter Selection and Adjustment
The selection and adjustment of parameters necessitate a strategic approach to achieve optimal results. By strategically evaluating the impact of each parameter on the model's performance, informed decisions can be made regarding their manipulation. This strategic discernment empowers practitioners to navigate the intricate landscape of hyperparameter optimization with precision, ultimately culminating in enhanced performance.
Unlocking AI Potential: Innovative Strategies for Enhancing Performance
In the quest to harness the full capabilities of artificial intelligence (AI), it becomes paramount to explore novel methodologies that can elevate its performance to unprecedented levels. This section delves into pioneering approaches aimed at maximizing the efficacy of AI systems, without solely relying on conventional means. By reimagining the paradigms of optimization and fine-tuning, we endeavor to unlock the latent potential ingrained within AI frameworks.
Revolutionizing Optimization Paradigms
The cornerstone of AI advancement lies in the ability to optimize its functionality seamlessly and intelligently. Traditional optimization techniques often fall short in capturing the intricacies of AI systems, necessitating a shift towards more dynamic and adaptive strategies. This subsection elucidates on the revolutionary methods devised to transcend the limitations of conventional optimization, paving the way for unparalleled performance enhancement in AI applications.
Fostering Innovation Through Adaptive Tuning
Central to the evolution of AI is the concept of continuous adaptation and refinement. Rather than adhering to static hyperparameter tuning approaches, this segment advocates for the integration of adaptive methodologies that can autonomously adjust and evolve in response to dynamic environments. By fostering innovation through adaptive tuning mechanisms, AI systems can achieve remarkable levels of versatility and robustness, thereby unlocking their true potential in diverse real-world scenarios.