In recent years, there has been a significant advancement in the field of Artificial Intelligence (AI) and Augmented Reality (AR). These technologies have become increasingly popular and have the potential to enhance virtual experiences in various fields such as gaming, education, healthcare, and...
Unlocking Knowledge - Harnessing Self-Supervised Learning for Unlabeled Data
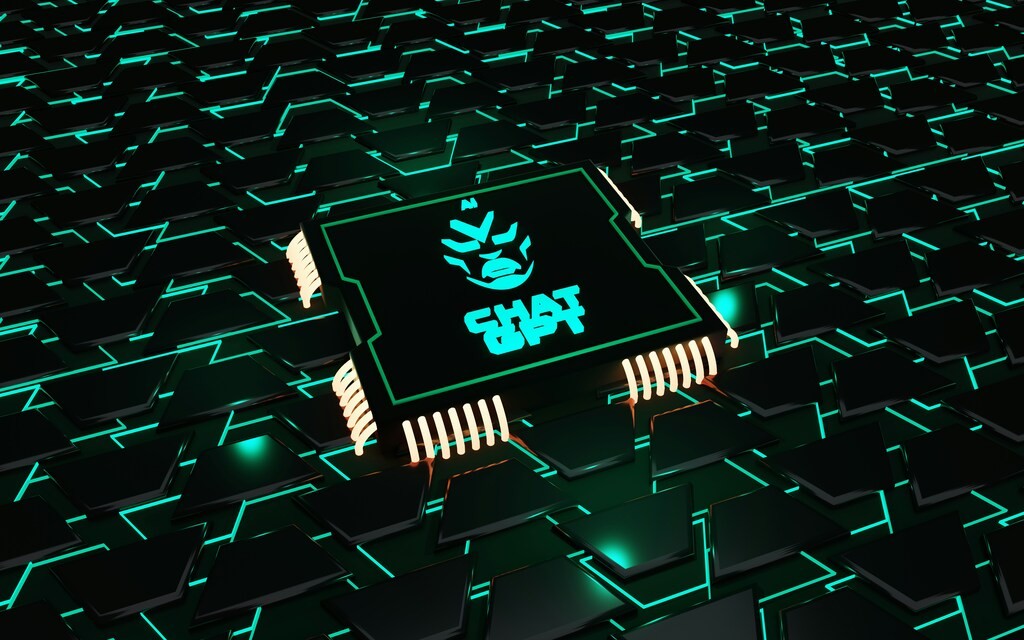
Embark on a journey where machines decipher the hidden intricacies of the world around them, not through explicit instruction, but by the sheer wealth of untapped resources at their disposal.
Imagine a realm where algorithms traverse uncharted territories of unprocessed content, extracting meaningful patterns and insights, unaided by human labels or annotations.
In this realm, the essence lies in the subtleties of the undetermined, where the absence of explicit guidance fosters a deeper understanding of the underlying structures inherent in the fabric of information.
This paradigm shift heralds a new era in artificial intelligence, where the focus shifts from prescribed tasks to the autonomous acquisition of knowledge through self-directed exploration.
Join us as we delve into the realm of autonomous cognition, where algorithms become adept at distilling wisdom from the unfiltered deluge of raw data, sculpting a landscape of insight from the unadulterated clay of information.
Unlocking Unlabeled Data: Insight into Autonomous Learning
In this section, we delve into the intricate realm of tapping into unmarked information sources through autonomous comprehension mechanisms. Rather than relying on external annotations or predefined labels, we explore the capacity of systems to independently extract meaningful insights from raw input. Through this process, machines autonomously navigate through the vast expanse of untagged datasets, deciphering patterns and structures without explicit guidance.
Unlocking |
Deciphering |
Unmarked |
Autonomous |
Unlocking |
Decoding |
Unlabeled |
Self-Governing |
Unveiling |
Interpreting |
Unannotated |
Independent |
Exploring |
Understanding |
Untagged |
Self-Directed |
By elucidating the mechanisms underpinning self-directed learning paradigms, we illuminate the pathways through which algorithms navigate the uncharted territory of raw data, extracting intricate insights and representations. Through this process, computational systems transcend the reliance on human-labeled datasets, fostering a realm of discovery where algorithms autonomously discern meaningful structures and relationships.
Discover the Power of Autonomous Discovery
In this section, we delve into the extraordinary capabilities of harnessing innate intelligence for unsupervised comprehension. By relinquishing the need for explicit guidance and tapping into the inherent structure of our environment, we unlock a realm of potential waiting to be unveiled.
Unlocking Unsupervised Insight: Through autonomous exploration, machines navigate the vast landscape of raw information, discerning patterns and correlations without the crutch of predefined labels. This autonomous discovery fosters a deeper understanding of the underlying principles governing our data-rich world.
Embracing Inherent Complexity: By embracing the intricacies inherent in our data, we transcend the limitations of traditional paradigms. Instead of relying on external annotations, we empower our models to distill complex relationships autonomously, paving the way for more robust and adaptable systems.
Unveiling Implicit Knowledge: Beneath the surface of unstructured data lies a wealth of implicit knowledge waiting to be unearthed. Through self-directed exploration, our models uncover latent insights, enriching their understanding of the world in a manner that transcends conventional supervision.
Empowering Adaptive Intelligence: By leveraging self-supervised approaches, we equip machines with the ability to adapt and evolve in dynamic environments. Freed from the shackles of explicit labels, our models embrace the fluidity of information, continuously refining their representations to reflect the ever-changing landscape of data.
Cultivating Resilient Systems: In the absence of labeled examples, our models learn to navigate uncertainty with grace, cultivating resilience in the face of ambiguity. This adaptability not only enhances performance in real-world scenarios but also lays the foundation for the next generation of intelligent systems.
Revolutionizing AI with Unlabeled Insights
In the realm of artificial intelligence, a paradigm shift is underway, driven by the untapped potential residing within unannotated datasets. This transformative approach harnesses the latent richness inherent in raw information, transcending traditional methods of model training. By delving into the depths of unlabelled reservoirs, AI systems are poised to unearth profound insights, fundamentally altering the landscape of machine intelligence.
The Power of Unmarked Discoveries
Within the vast expanse of unmarked data lie hidden treasures awaiting discovery. Liberated from the constraints of explicit annotations, AI systems embark on a journey of exploration, extracting nuanced patterns and subtle correlations. This liberation from predefined labels fosters a deeper understanding of the underlying structure of data, empowering models to generalize with unprecedented accuracy.
Unlocking Potential through Unsupervised Endeavors
By embracing the uncharted territories of unlabelled datasets, AI enters a realm of self-discovery and adaptability. Through unsupervised methodologies, algorithms autonomously unravel the intricacies of raw data, distilling meaningful representations without the need for external guidance. This autonomy not only enhances efficiency but also cultivates resilience, equipping AI systems to thrive in dynamic and diverse environments.
Unraveling the Secrets of Autonomous Insight Generation
In this section, we embark on a journey to delve into the enigmatic realm of autonomous insight generation, a process shrouded in mystery yet brimming with potential. By harnessing the latent patterns within unannotated information, this methodology unveils a clandestine pathway to knowledge acquisition without the traditional scaffolding of explicit guidance.
Deciphering Intrinsic Signals: At the core of this approach lies the art of deciphering intrinsic signals concealed within raw data streams, transcending the need for external supervision. Through a meticulous exploration of the underlying structures and relationships, these concealed cues serve as beacons guiding the system towards a profound understanding of the data landscape.
Unlocking Latent Semantics: Beyond surface-level observations, this paradigm endeavors to unlock the latent semantics embedded within the unadorned information canvas. By discerning subtle contextual nuances and semantic correlations, the model navigates the intricate web of data, unraveling its hidden narrative with unparalleled finesse.
Empowering Adaptive Abstraction: Through iterative refinement, the system attains a mastery of adaptive abstraction, distilling complex concepts into concise representations. This dynamic process of abstraction empowers the model to encapsulate the essence of diverse data modalities, fostering a versatile framework for subsequent inference and understanding.
Embarking on a Quest for Unveiling: As we embark on this quest for unveiling, we embrace the challenge of unraveling the intricacies of autonomous insight generation. Through an amalgamation of computational prowess and intuitive exploration, we aspire to unlock the secrets that lie dormant within the unexplored realms of unannotated data.