In recent years, there has been a significant advancement in the field of Artificial Intelligence (AI) and Augmented Reality (AR). These technologies have become increasingly popular and have the potential to enhance virtual experiences in various fields such as gaming, education, healthcare, and...
Unlocking the Black Box - How Interpretable Machine Learning Offers Insights into AI Predictions
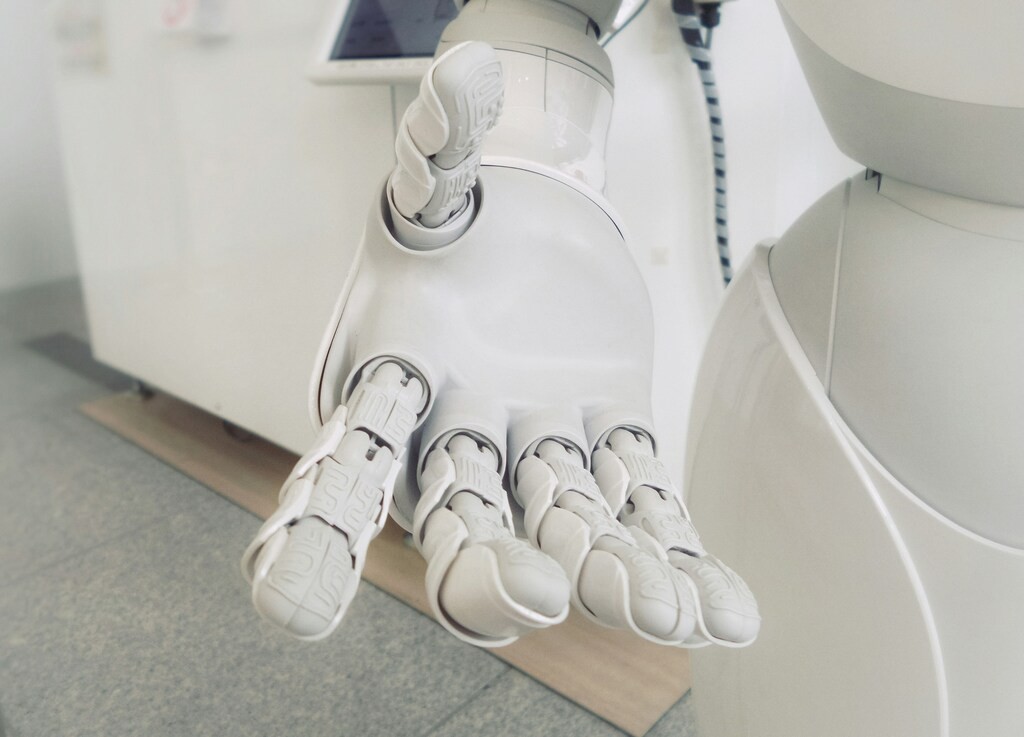
In the realm of computational intelligence, the quest for transparency in algorithmic outcomes represents a pivotal pursuit. Unveiling the inner workings of AI systems and elucidating the rationale behind their conclusions stands as a cornerstone of modern technological ethics and accountability. This imperative journey involves deciphering the cryptic mechanisms driving automated insights, paving the way towards comprehensible and justifiable AI outputs.
Peering Beyond the Veil: Within the labyrinth of artificial cognition, lies the challenge of discerning the invisible threads weaving through complex data landscapes. Here, the aspiration is not merely to unveil the outcomes but to traverse the cognitive pathways leading to these deductions. This endeavor beckons towards a paradigm shift, from opaque predictions to transparent deliberations, fostering trust and fostering a deeper understanding of AI's decision-making prowess.
Democratizing Knowledge: The democratization of insight emerges as a rallying cry in the discourse surrounding AI accountability. Empowering users with the ability to decipher the cryptic language of algorithms fosters a culture of informed decision-making and mitigates the risks of blind reliance on machine-driven outcomes. Through accessible explanations, AI transcends its enigmatic facade, transforming into a tool for empowerment and enlightenment.
Understanding Insightful AI Forecasts
In delving into the realm of comprehensible computational intelligence, one must embark on a journey to decipher the inner workings of algorithms designed to shed light on opaque forecasts. This segment endeavors to navigate the intricate landscape of AI models engineered to illuminate predictions through transparent mechanisms.
The Essence of Transparent Prediction
At its core, the essence of lucid AI prognostication lies in its capacity to demystify the enigmatic processes that underpin predictive outcomes. Through elucidating the intricate pathways traversed by algorithms, stakeholders gain invaluable insights into the rationale behind each forecast, fostering trust and confidence in the computational decision-making apparatus.
Unraveling the Veil of Predictive Uncertainty
Beyond mere elucidation, the pursuit of interpretable AI necessitates an unwavering commitment to unraveling the veil of uncertainty that often shrouds machine-driven forecasts. By providing clarity amidst ambiguity, interpretable models pave the way for informed decision-making, empowering users to navigate the complexities of tomorrow with foresight and assurance.
The Significance of Transparency in AI
In the realm of artificial intelligence, the clarity and openness of algorithms play a pivotal role in fostering trust and comprehension among users. Unveiling the inner workings of AI systems not only enhances accountability but also empowers individuals to comprehend the rationale behind decisions made by these sophisticated systems.
|
|
Approaches for Explanatory Models in AI
In this section, we delve into various methodologies aimed at enhancing the transparency and intelligibility of artificial intelligence systems. Understanding the inner workings of AI models is crucial for fostering trust and facilitating their integration into real-world applications. By exploring diverse strategies and techniques, we aim to shed light on the mechanisms behind AI decision-making processes.
1. Transparency through Simplification
One avenue for enhancing the interpretability of AI models involves simplifying their complexities. This approach entails distilling intricate algorithms into more understandable representations, allowing stakeholders to grasp the underlying logic without delving into intricate technicalities. Techniques such as feature importance ranking, model distillation, and rule extraction enable the creation of simplified versions of AI systems, offering insights into the factors driving their predictions.
2. Intuitive Visualization Techniques
Visual representations play a pivotal role in elucidating AI predictions and fostering comprehension among non-technical audiences. Leveraging intuitive visualization techniques, such as decision trees, saliency maps, and activation maximization, enables stakeholders to gain insights into how AI models process information and arrive at specific outcomes. By translating abstract concepts into visual forms, these methods facilitate intuitive understanding and promote transparency in AI-driven decision-making processes.
- Feature importance ranking
- Model distillation
- Rule extraction
- Decision trees
- Saliency maps
- Activation maximization
By employing a combination of these approaches and techniques, stakeholders can gain deeper insights into the inner workings of AI models, thereby fostering trust and confidence in their decision-making capabilities.
Techniques for Model Transparency
In the realm of understanding AI predictions, attaining clarity regarding the inner workings of models becomes paramount. Herein lies the exploration of methodologies aimed at illuminating the opaque processes governing AI outcomes.
- Feature Importance Analysis: Unveiling the significance of input variables in model decision-making through various statistical measures.
- Partial Dependence Plots: Visualizing the relationship between specific features and model predictions, allowing for nuanced comprehension of their influence.
- Local Interpretable Model-agnostic Explanations (LIME): Employing local surrogate models to approximate the behavior of complex models for individual predictions, offering insights into their reasoning.
- Shapley Values: Assigning credit to each feature for a particular prediction, discerning their respective contributions to the model's output.
- Decision Trees and Rule Extraction: Constructing interpretable decision rules mimicking the behavior of black-box models, facilitating intuitive understanding of their decision logic.
These techniques serve as indispensable tools in demystifying the enigmatic nature of AI predictions, fostering trust and comprehension in AI systems across various domains.
Applications of Transparent AI Insights
Exploring the practical domains where comprehensible AI insights find their footing unveils a spectrum of impactful applications. By demystifying the inner workings of AI models and shedding light on their decision-making processes, transparent AI insights pave the way for enhanced trust and adoption across diverse sectors.
Enhanced Medical Diagnostics
- Transparent AI insights empower medical professionals to decipher the rationale behind diagnostic recommendations, fostering collaboration between man and machine in the realm of healthcare.
- These insights can elucidate complex medical predictions, offering clinicians actionable information to refine treatment plans and optimize patient outcomes.
Financial Risk Assessment and Management
- Within the financial sector, transparent AI insights serve as a guiding compass, allowing stakeholders to navigate through intricate risk landscapes with confidence.
- By providing intelligible explanations for AI-driven predictions, financial institutions can bolster regulatory compliance and cultivate a deeper understanding of market dynamics.
Across industries, from retail to transportation, the integration of transparent AI insights heralds a new era of informed decision-making, where stakeholders leverage the power of AI with clarity and conviction.