In recent years, there has been a significant advancement in the field of Artificial Intelligence (AI) and Augmented Reality (AR). These technologies have become increasingly popular and have the potential to enhance virtual experiences in various fields such as gaming, education, healthcare, and...
Unlocking Visual Representation Learning - Harnessing Features Unaided by Labels
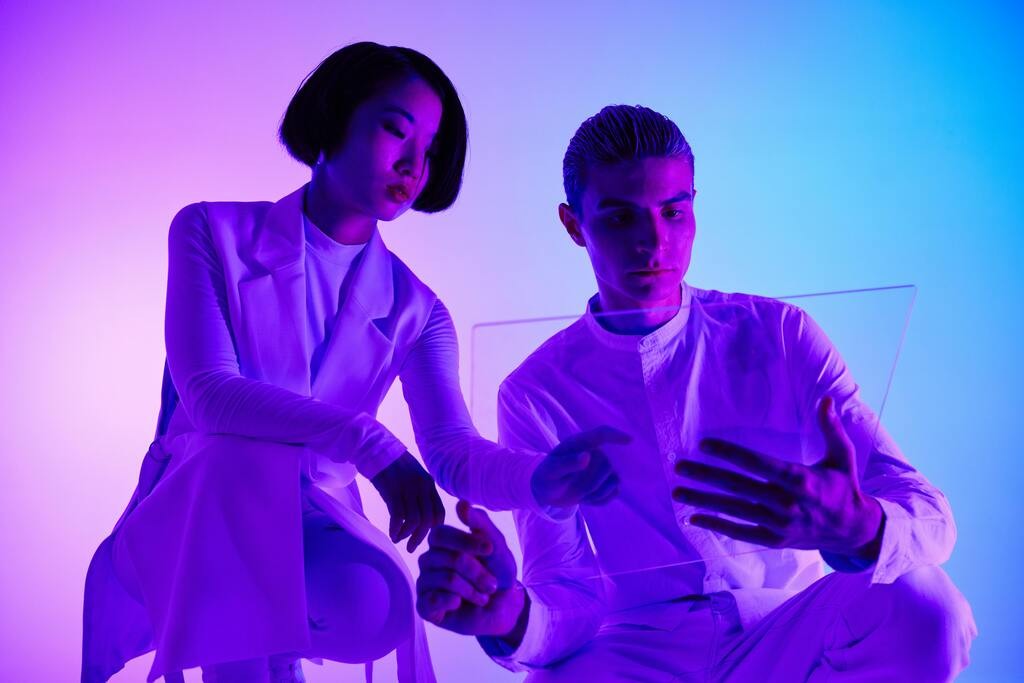
Embark on a journey through the realm of cognition, where machines evolve to interpret the visual universe with unprecedented acumen. Imagine a landscape where understanding transcends the confines of explicit guidance, where intelligence thrives on the essence of self-discovery. In this narrative, we delve into the realm of autonomous comprehension, where systems embark on a quest to discern patterns and extract meaning from uncharted visual data. This voyage is not guided by traditional labels or predefined paths; instead, it embraces the ethos of self-supervision, where learning unfurls organically in the absence of explicit directives.
Engage with a paradigm shift that revolutionizes the landscape of computational perception. Bid farewell to the shackles of labeled datasets and predetermined annotations. Here, in the crucible of self-directed learning, algorithms forge their understanding, weaving intricate webs of knowledge from the raw fabric of visual stimuli. This isn't merely a quest for accuracy; it's a relentless pursuit of insight, where algorithms evolve akin to sentient beings, honing their perceptual prowess with each encounter.
Illuminate the pathways of innovation as we unravel the tapestry of unsupervised visual representation learning. Witness the emergence of a new era, where data becomes not just a resource, but a fountain of wisdom waiting to be tapped. Through the lens of self-guided exploration, we unveil the latent potential residing within every pixel, transforming the mundane into the extraordinary. Here, in the absence of explicit labels, lies the crucible where intelligence is tempered and understanding is forged.
Understanding Autonomous Visual Encoding Acquisition
In this segment, we delve into the realm of self-driven comprehension in visual data encoding acquisition. Here, we embark on a journey to grasp the essence of autonomous knowledge extraction, unbound by conventional instructional paradigms. Through this exploration, we uncover the mechanisms underlying the autonomous generation of visual representations, liberated from explicit directives or predefined categorizations.
The Essence of Unsupervised Insight Generation
Within this domain, the crux lies in the innate capacity of systems to autonomously distill meaningful insights from visual stimuli, unfettered by external annotations or supervision. Here, the emphasis shifts from traditional learning paradigms towards a more organic process of encoding acquisition, driven by intrinsic motivations and the inherent structure of the data itself.
Unlocking the Intricacies of Implicit Knowledge Assimilation
This section delves into the intricacies of implicit knowledge assimilation, wherein systems navigate the vast landscape of visual data to extract latent patterns and structures. Through this lens, we unravel the mechanisms through which autonomous entities discern relevant features and construct coherent representations, fostering a deeper understanding of the underlying processes driving self-supervised visual encoding acquisition.
The Concept and Importance of Autonomous Learning
In this section, we delve into the fundamental principles and significance of autonomous learning, a method gaining traction in contemporary research landscapes. Autonomous learning represents a paradigm shift in how we approach the acquisition of knowledge, emphasizing self-directed exploration and discovery over traditional pedagogical frameworks. It embodies the notion of cognitive independence, empowering systems to extract meaningful insights from raw data without explicit guidance or supervision.
At its core, autonomous learning harnesses intrinsic properties of data to drive feature extraction and pattern recognition. By leveraging inherent structures and relationships within the data itself, autonomous systems can autonomously uncover relevant information and distill complex representations without reliance on annotated labels or external cues. This transformative approach not only circumvents the need for extensive human intervention but also holds the potential to unlock deeper layers of understanding from vast and unstructured datasets.
- Empowerment Through Autonomy: Autonomous learning liberates models from the constraints of labeled data, fostering a sense of self-reliance and adaptability. By encouraging systems to navigate and make sense of data autonomously, this approach cultivates resilience and versatility in handling diverse tasks and domains.
- Unveiling Latent Structures: By delving beneath the surface of raw data, autonomous learning unveils latent structures and intricate patterns that may elude traditional supervised methods. This innate ability to discern meaningful representations enables systems to capture the essence of data more comprehensively, facilitating nuanced decision-making and insightful analysis.
- Scalability and Generalization: Autonomous learning exhibits remarkable scalability and generalization capabilities, transcending the limitations imposed by labeled datasets. By harnessing the intrinsic richness of unannotated data, autonomous systems can adapt and generalize across diverse contexts and environments, laying the groundwork for robust and transferable knowledge representations.
Overall, the concept of autonomous learning heralds a new era of discovery and innovation, where machines can autonomously navigate the vast seas of data, extracting pearls of wisdom without the need for external guidance. By embracing autonomy as a cornerstone of learning, we pave the way for more resilient, adaptable, and insightful artificial intelligence systems.
Key Strategies and Approaches in Visual Feature Acquisition
Exploring the landscape of visual cognition, we delve into the fundamental methodologies and principles driving the acquisition of rich visual features, transcending the traditional paradigms of supervised annotation. Within this domain, an array of innovative techniques and strategies emerge, aiming to harness the latent potential within unannotated data.
Central to this endeavor is the notion of autonomous discovery, where algorithms are tasked with discerning salient patterns and structures from raw visual input. Leveraging intrinsic cues and contextual relationships, these methodologies strive to distill meaningful representations without explicit guidance.
Moreover, the pursuit of discriminative capability stands as a cornerstone in this pursuit. Through various mechanisms such as contrastive learning and pretext tasks, models endeavor to extract features that capture the essence of visual semantics, facilitating downstream tasks with enhanced efficacy.
Furthermore, the amalgamation of diverse data modalities and multi-modal fusion techniques introduces a nuanced dimension to feature acquisition. By synthesizing information from disparate sources, models can attain a holistic understanding of the visual world, transcending the confines of individual data domains.
In essence, the exploration of key techniques and approaches in visual feature acquisition embodies a paradigm shift in machine perception, heralding a future where algorithms possess the innate ability to distill intricate visual semantics from unstructured data streams.
Applications and Implications in Computer Vision and Beyond
In this section, we delve into the practical applications and broader implications of harnessing autonomous methods for visual feature extraction, extending far beyond the conventional realms of image processing and cognition. By leveraging self-guided techniques for understanding and extracting meaningful patterns from visual data, we unlock a plethora of possibilities across various domains.
Revolutionizing Visual Understanding: The utilization of autonomous mechanisms for discerning intricate details within images fundamentally reshapes how we perceive and interpret visual information. Through this paradigm shift, we not only enhance traditional computer vision tasks such as object recognition and scene understanding but also pave the way for novel applications in fields like autonomous vehicles, medical imaging, and satellite analysis.
Breaking the Label Dependency: By divorcing reliance on labeled datasets, we transcend the constraints imposed by labor-intensive annotation processes. This liberation empowers systems to autonomously learn and adapt to diverse visual contexts, fostering robustness and scalability in real-world scenarios. Beyond computer vision, this approach catalyzes advancements in domains where labeled data is scarce or costly to obtain, such as robotics, environmental monitoring, and industrial automation.
Enabling Cross-Domain Insights: The insights garnered from self-directed feature learning transcend disciplinary boundaries, permeating into fields beyond traditional computer science realms. From aiding historians in analyzing ancient artifacts to assisting biologists in understanding intricate biological structures, the applications span a diverse array of disciplines. This interdisciplinary synergy amplifies the impact of autonomous visual representation learning, ushering in a new era of knowledge discovery and innovation.